Derivation of CDF of a function that results in an exponential distributionUse Empirical CDF vs Distribution CDF?CDF of conditional distributioncumulative distribution function , cdf problemCDF of distribution $A+B$Deriving Density Function (pdf) from Distribution Function (cdf)Distribution function terminology (PDF, CDF, PMF, etc.)Strange results from t-student cdf with “Abramowitz” approximationCDF of a Tweedie DistributionCDF of multiple exponential random variablesWhat is the problem in my CDF derivation?
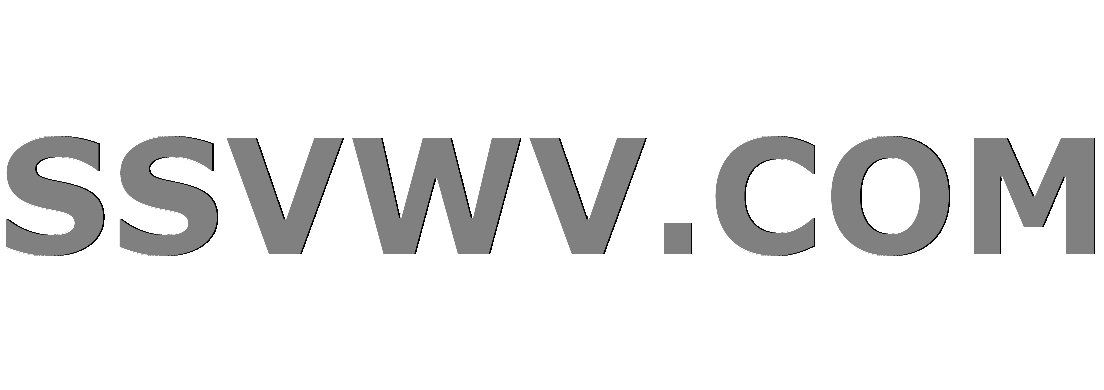
Multi tool use
Having some issue with notation in a Hilbert space
What is this plant I saw for sale at a Romanian farmer's market?
How to avoid offending original culture when making conculture inspired from original
Fill the maze with a wall-following Snake until it gets stuck
How to recover a single blank shot from a film camera
How to make a villain when your PCs are villains?
Query nodes and attributes of parent ways
King or Queen-Which piece is which?
How can I detect if I'm in a subshell?
Basic power tool set for Home repair and simple projects
Is a sequel allowed to start before the end of the first book?
How do credit card companies know what type of business I'm paying for?
Should I email my professor to clear up a (possibly very irrelevant) awkward misunderstanding?
Scaling an object to change its key
Are there any individual aliens that have gained superpowers in the Marvel universe?
Can a character with the Polearm Master feat make an opportunity attack against an invisible creature that enters their reach?
A medieval book with a redhead girl as a main character who allies with vampires and werewolves against scientific opposition
Fibonacci sequence and other metallic sequences emerged in the form of fractions
How can I ping multiple IP addresses at the same time?
Derivation of CDF of a function that results in an exponential distribution
How "fast" do astronomical events occur?
Print the new site header
What is the precise meaning of "подсел на мак"?
Would a 7805 5v regulator drain a 9v battery?
Derivation of CDF of a function that results in an exponential distribution
Use Empirical CDF vs Distribution CDF?CDF of conditional distributioncumulative distribution function , cdf problemCDF of distribution $A+B$Deriving Density Function (pdf) from Distribution Function (cdf)Distribution function terminology (PDF, CDF, PMF, etc.)Strange results from t-student cdf with “Abramowitz” approximationCDF of a Tweedie DistributionCDF of multiple exponential random variablesWhat is the problem in my CDF derivation?
.everyoneloves__top-leaderboard:empty,.everyoneloves__mid-leaderboard:empty,.everyoneloves__bot-mid-leaderboard:empty margin-bottom:0;
$begingroup$
I was looking through wiki's treatment on the title topic in https://en.wikipedia.org/wiki/Random_variable and am completely stumped on this particular section:
There are several specifics that elude me.
- How is the following progression derived
$$Y = log(1 + e^-X) Longrightarrow F_Y(y) = Pr( log(1+e^-X) le y )$$
and then the next step
$$Pr( log(1+e^-X) le y) = Pr( X ge -log(e^y - 1) )$$
Thx for filling in the blanks.
cdf
$endgroup$
add a comment |
$begingroup$
I was looking through wiki's treatment on the title topic in https://en.wikipedia.org/wiki/Random_variable and am completely stumped on this particular section:
There are several specifics that elude me.
- How is the following progression derived
$$Y = log(1 + e^-X) Longrightarrow F_Y(y) = Pr( log(1+e^-X) le y )$$
and then the next step
$$Pr( log(1+e^-X) le y) = Pr( X ge -log(e^y - 1) )$$
Thx for filling in the blanks.
cdf
$endgroup$
$begingroup$
Hint: By definition, for each real number $alpha$, the value of tthe CDF $F_Y$ at $alpha$, usually expressed as $F_Y(alpha)$, equals the probability that $Y$ is smaller than $alpha$. So, knowing this, can you figure out for yourself why the implication $$Y = log(1 + e^-X) Longrightarrow F_Y(y) = Pr(Y leq y) = Pr( log(1+e^-X) le y )$$ holds??
$endgroup$
– Dilip Sarwate
5 hours ago
$begingroup$
In what I wrote above, for "$Y$ is smaller than $alpha$", please substitute "$Y$ is no larger than $alpha$".
$endgroup$
– Dilip Sarwate
5 hours ago
add a comment |
$begingroup$
I was looking through wiki's treatment on the title topic in https://en.wikipedia.org/wiki/Random_variable and am completely stumped on this particular section:
There are several specifics that elude me.
- How is the following progression derived
$$Y = log(1 + e^-X) Longrightarrow F_Y(y) = Pr( log(1+e^-X) le y )$$
and then the next step
$$Pr( log(1+e^-X) le y) = Pr( X ge -log(e^y - 1) )$$
Thx for filling in the blanks.
cdf
$endgroup$
I was looking through wiki's treatment on the title topic in https://en.wikipedia.org/wiki/Random_variable and am completely stumped on this particular section:
There are several specifics that elude me.
- How is the following progression derived
$$Y = log(1 + e^-X) Longrightarrow F_Y(y) = Pr( log(1+e^-X) le y )$$
and then the next step
$$Pr( log(1+e^-X) le y) = Pr( X ge -log(e^y - 1) )$$
Thx for filling in the blanks.
cdf
cdf
edited 6 hours ago
Michael Hardy
4,4541430
4,4541430
asked 8 hours ago
javadbajavadba
240214
240214
$begingroup$
Hint: By definition, for each real number $alpha$, the value of tthe CDF $F_Y$ at $alpha$, usually expressed as $F_Y(alpha)$, equals the probability that $Y$ is smaller than $alpha$. So, knowing this, can you figure out for yourself why the implication $$Y = log(1 + e^-X) Longrightarrow F_Y(y) = Pr(Y leq y) = Pr( log(1+e^-X) le y )$$ holds??
$endgroup$
– Dilip Sarwate
5 hours ago
$begingroup$
In what I wrote above, for "$Y$ is smaller than $alpha$", please substitute "$Y$ is no larger than $alpha$".
$endgroup$
– Dilip Sarwate
5 hours ago
add a comment |
$begingroup$
Hint: By definition, for each real number $alpha$, the value of tthe CDF $F_Y$ at $alpha$, usually expressed as $F_Y(alpha)$, equals the probability that $Y$ is smaller than $alpha$. So, knowing this, can you figure out for yourself why the implication $$Y = log(1 + e^-X) Longrightarrow F_Y(y) = Pr(Y leq y) = Pr( log(1+e^-X) le y )$$ holds??
$endgroup$
– Dilip Sarwate
5 hours ago
$begingroup$
In what I wrote above, for "$Y$ is smaller than $alpha$", please substitute "$Y$ is no larger than $alpha$".
$endgroup$
– Dilip Sarwate
5 hours ago
$begingroup$
Hint: By definition, for each real number $alpha$, the value of tthe CDF $F_Y$ at $alpha$, usually expressed as $F_Y(alpha)$, equals the probability that $Y$ is smaller than $alpha$. So, knowing this, can you figure out for yourself why the implication $$Y = log(1 + e^-X) Longrightarrow F_Y(y) = Pr(Y leq y) = Pr( log(1+e^-X) le y )$$ holds??
$endgroup$
– Dilip Sarwate
5 hours ago
$begingroup$
Hint: By definition, for each real number $alpha$, the value of tthe CDF $F_Y$ at $alpha$, usually expressed as $F_Y(alpha)$, equals the probability that $Y$ is smaller than $alpha$. So, knowing this, can you figure out for yourself why the implication $$Y = log(1 + e^-X) Longrightarrow F_Y(y) = Pr(Y leq y) = Pr( log(1+e^-X) le y )$$ holds??
$endgroup$
– Dilip Sarwate
5 hours ago
$begingroup$
In what I wrote above, for "$Y$ is smaller than $alpha$", please substitute "$Y$ is no larger than $alpha$".
$endgroup$
– Dilip Sarwate
5 hours ago
$begingroup$
In what I wrote above, for "$Y$ is smaller than $alpha$", please substitute "$Y$ is no larger than $alpha$".
$endgroup$
– Dilip Sarwate
5 hours ago
add a comment |
3 Answers
3
active
oldest
votes
$begingroup$
Introduction. The so-called "CDF method" is one way to find the distribution of a
the transformation $Y = g(X)$ of a random variable $X$ with a known CDF.
Let's look at a simpler example first: Suppose $X sim mathsfUniv(0,1)$ and find the CDF of $Y = g(X) = sqrtX.$ The support of $X$ is $(0,1)$ and it is clear that the support of $Y$ will also be $(0,1).$
The CDF of $X$ is $F_X(x) = x,$ for $x in (0,1).$ Then the CDF of $Y$ is
$$P(Y le y) = P(g(X) le y) = P(sqrtX le y) = P(X le y^2) = y^2,$$
for $y in (0,1).$ The last step uses $F_X(x) = P(X le x) = x,$ where $y^2 = x.$ Thus the PDF of $Y$ is $f_Y(y) = F_Y^prime(y) = dy^2/dy = 2y,$ which
we recognize as the PDF of $mathsfBeta(2,1).$
Illustrating this with a random sample of $n = 10^5$ observations $X_i$ from $mathsfUnif(0,1),$ we have the following results (in R):
set.seed(615)
x = runif(10^5, 0, 1); y = sqrt(x)
par(mfrow=c(1,2))
hist(x, prob=T, col="skyblue2", main="X ~ UNIF(0,1)")
curve(dunif(x, 0, 1), add=T, n=10001, lwd=2, col="brown")
hist(y, prob=T, col="skyblue2", main="Y ~ BETA(2,1)")
curve(dbeta(x, 2, 1), add=T, n=10001, lwd=2, col="brown")
par(mfrow=c(1,1))
Your Question. Now let's do a similar procedure for $X$ with CDF $F_X(x) = P(X le x)
= (1 + e^-x)^-theta,$ for $theta > 0$ and the transformation
$Y = g(X) = log(1+e^-X),$ which has support $(0, infty).$
Using the CDF method again, we have:
$$F_Y(y) = P(Yle y) = P(log(1 + e^-X)le y) = P(1+e^-X le e^y)\
=P(e^-X le e^y - 1) = P(-X le log(e^y -1))\ = P(X ge -log(e^y -1)) = cdots,$$
So, $F_y(y) = 1-e^-theta y,$ for $y > 0,$ as claimed.
We illustrate with a random sample of $n = 10^5$ observations from the
original logistic distribution with $theta = 1.$ This distribution can be sampled in terms of standard uniform distributions as shown in the R code;
see Wikipedia, second bullet under Related Distributions.
set.seed(2019)
u = runif(10^5); x = log(u) - log(1-u)
y = log(1 + exp(-x))
par(mfrow=c(1,2))
hist(x, prob=T, br=30, ylim=c(0,.25), col="skyblue2", main="Logistic")
curve(exp(-x)/(1+exp(-x))^2, add=T, lwd=2, col="brown")
hist(y, prob=T, ylim=c(0,1), col="skyblue2", main="Exponential")
curve(dexp(x,1), add=T, lwd=2, n=10001, col="brown")
par(mfrow=c(1,1))
$endgroup$
add a comment |
$begingroup$
beginalign
& log(1+e^-X) le y \[12pt]
& 1+e^-X le e^y \
& textsince log text and exp \
& textare increasing functions \[12pt]
& e^-X le e^y - 1 \[12pt]
& -X le log(e^y - 1) \
& textsince log text and exp \
& textare increasing functions \[12pt]
& X ge -log(e^y - 1)
endalign
$endgroup$
add a comment |
$begingroup$
Question 1:
By definition, FY(y) = Pr(Y<=y)
And because we know that Y=log(1+e^−X)
Then FY(y) = Pr(log(1+e^−X) <= y)
Question 2:
All they're doing there is starting with log(1+e^−X)≤y
and simply solving for X
which entails, first doing e^ on both sides:
1+e^−X ≤ e^y
then sending the 1 to the right:
e^−X ≤ e^y - 1
then doing log on both sides:
−X ≤ log(e^y - 1)
and finally multiplying both sides by -1, which changes the direction of the ≤
So you end up with:
X≥−log(e^y−1)
which is what they got
New contributor
Dave is a new contributor to this site. Take care in asking for clarification, commenting, and answering.
Check out our Code of Conduct.
$endgroup$
add a comment |
Your Answer
StackExchange.ready(function()
var channelOptions =
tags: "".split(" "),
id: "65"
;
initTagRenderer("".split(" "), "".split(" "), channelOptions);
StackExchange.using("externalEditor", function()
// Have to fire editor after snippets, if snippets enabled
if (StackExchange.settings.snippets.snippetsEnabled)
StackExchange.using("snippets", function()
createEditor();
);
else
createEditor();
);
function createEditor()
StackExchange.prepareEditor(
heartbeatType: 'answer',
autoActivateHeartbeat: false,
convertImagesToLinks: false,
noModals: true,
showLowRepImageUploadWarning: true,
reputationToPostImages: null,
bindNavPrevention: true,
postfix: "",
imageUploader:
brandingHtml: "Powered by u003ca class="icon-imgur-white" href="https://imgur.com/"u003eu003c/au003e",
contentPolicyHtml: "User contributions licensed under u003ca href="https://creativecommons.org/licenses/by-sa/3.0/"u003ecc by-sa 3.0 with attribution requiredu003c/au003e u003ca href="https://stackoverflow.com/legal/content-policy"u003e(content policy)u003c/au003e",
allowUrls: true
,
onDemand: true,
discardSelector: ".discard-answer"
,immediatelyShowMarkdownHelp:true
);
);
Sign up or log in
StackExchange.ready(function ()
StackExchange.helpers.onClickDraftSave('#login-link');
);
Sign up using Google
Sign up using Facebook
Sign up using Email and Password
Post as a guest
Required, but never shown
StackExchange.ready(
function ()
StackExchange.openid.initPostLogin('.new-post-login', 'https%3a%2f%2fstats.stackexchange.com%2fquestions%2f413187%2fderivation-of-cdf-of-a-function-that-results-in-an-exponential-distribution%23new-answer', 'question_page');
);
Post as a guest
Required, but never shown
3 Answers
3
active
oldest
votes
3 Answers
3
active
oldest
votes
active
oldest
votes
active
oldest
votes
$begingroup$
Introduction. The so-called "CDF method" is one way to find the distribution of a
the transformation $Y = g(X)$ of a random variable $X$ with a known CDF.
Let's look at a simpler example first: Suppose $X sim mathsfUniv(0,1)$ and find the CDF of $Y = g(X) = sqrtX.$ The support of $X$ is $(0,1)$ and it is clear that the support of $Y$ will also be $(0,1).$
The CDF of $X$ is $F_X(x) = x,$ for $x in (0,1).$ Then the CDF of $Y$ is
$$P(Y le y) = P(g(X) le y) = P(sqrtX le y) = P(X le y^2) = y^2,$$
for $y in (0,1).$ The last step uses $F_X(x) = P(X le x) = x,$ where $y^2 = x.$ Thus the PDF of $Y$ is $f_Y(y) = F_Y^prime(y) = dy^2/dy = 2y,$ which
we recognize as the PDF of $mathsfBeta(2,1).$
Illustrating this with a random sample of $n = 10^5$ observations $X_i$ from $mathsfUnif(0,1),$ we have the following results (in R):
set.seed(615)
x = runif(10^5, 0, 1); y = sqrt(x)
par(mfrow=c(1,2))
hist(x, prob=T, col="skyblue2", main="X ~ UNIF(0,1)")
curve(dunif(x, 0, 1), add=T, n=10001, lwd=2, col="brown")
hist(y, prob=T, col="skyblue2", main="Y ~ BETA(2,1)")
curve(dbeta(x, 2, 1), add=T, n=10001, lwd=2, col="brown")
par(mfrow=c(1,1))
Your Question. Now let's do a similar procedure for $X$ with CDF $F_X(x) = P(X le x)
= (1 + e^-x)^-theta,$ for $theta > 0$ and the transformation
$Y = g(X) = log(1+e^-X),$ which has support $(0, infty).$
Using the CDF method again, we have:
$$F_Y(y) = P(Yle y) = P(log(1 + e^-X)le y) = P(1+e^-X le e^y)\
=P(e^-X le e^y - 1) = P(-X le log(e^y -1))\ = P(X ge -log(e^y -1)) = cdots,$$
So, $F_y(y) = 1-e^-theta y,$ for $y > 0,$ as claimed.
We illustrate with a random sample of $n = 10^5$ observations from the
original logistic distribution with $theta = 1.$ This distribution can be sampled in terms of standard uniform distributions as shown in the R code;
see Wikipedia, second bullet under Related Distributions.
set.seed(2019)
u = runif(10^5); x = log(u) - log(1-u)
y = log(1 + exp(-x))
par(mfrow=c(1,2))
hist(x, prob=T, br=30, ylim=c(0,.25), col="skyblue2", main="Logistic")
curve(exp(-x)/(1+exp(-x))^2, add=T, lwd=2, col="brown")
hist(y, prob=T, ylim=c(0,1), col="skyblue2", main="Exponential")
curve(dexp(x,1), add=T, lwd=2, n=10001, col="brown")
par(mfrow=c(1,1))
$endgroup$
add a comment |
$begingroup$
Introduction. The so-called "CDF method" is one way to find the distribution of a
the transformation $Y = g(X)$ of a random variable $X$ with a known CDF.
Let's look at a simpler example first: Suppose $X sim mathsfUniv(0,1)$ and find the CDF of $Y = g(X) = sqrtX.$ The support of $X$ is $(0,1)$ and it is clear that the support of $Y$ will also be $(0,1).$
The CDF of $X$ is $F_X(x) = x,$ for $x in (0,1).$ Then the CDF of $Y$ is
$$P(Y le y) = P(g(X) le y) = P(sqrtX le y) = P(X le y^2) = y^2,$$
for $y in (0,1).$ The last step uses $F_X(x) = P(X le x) = x,$ where $y^2 = x.$ Thus the PDF of $Y$ is $f_Y(y) = F_Y^prime(y) = dy^2/dy = 2y,$ which
we recognize as the PDF of $mathsfBeta(2,1).$
Illustrating this with a random sample of $n = 10^5$ observations $X_i$ from $mathsfUnif(0,1),$ we have the following results (in R):
set.seed(615)
x = runif(10^5, 0, 1); y = sqrt(x)
par(mfrow=c(1,2))
hist(x, prob=T, col="skyblue2", main="X ~ UNIF(0,1)")
curve(dunif(x, 0, 1), add=T, n=10001, lwd=2, col="brown")
hist(y, prob=T, col="skyblue2", main="Y ~ BETA(2,1)")
curve(dbeta(x, 2, 1), add=T, n=10001, lwd=2, col="brown")
par(mfrow=c(1,1))
Your Question. Now let's do a similar procedure for $X$ with CDF $F_X(x) = P(X le x)
= (1 + e^-x)^-theta,$ for $theta > 0$ and the transformation
$Y = g(X) = log(1+e^-X),$ which has support $(0, infty).$
Using the CDF method again, we have:
$$F_Y(y) = P(Yle y) = P(log(1 + e^-X)le y) = P(1+e^-X le e^y)\
=P(e^-X le e^y - 1) = P(-X le log(e^y -1))\ = P(X ge -log(e^y -1)) = cdots,$$
So, $F_y(y) = 1-e^-theta y,$ for $y > 0,$ as claimed.
We illustrate with a random sample of $n = 10^5$ observations from the
original logistic distribution with $theta = 1.$ This distribution can be sampled in terms of standard uniform distributions as shown in the R code;
see Wikipedia, second bullet under Related Distributions.
set.seed(2019)
u = runif(10^5); x = log(u) - log(1-u)
y = log(1 + exp(-x))
par(mfrow=c(1,2))
hist(x, prob=T, br=30, ylim=c(0,.25), col="skyblue2", main="Logistic")
curve(exp(-x)/(1+exp(-x))^2, add=T, lwd=2, col="brown")
hist(y, prob=T, ylim=c(0,1), col="skyblue2", main="Exponential")
curve(dexp(x,1), add=T, lwd=2, n=10001, col="brown")
par(mfrow=c(1,1))
$endgroup$
add a comment |
$begingroup$
Introduction. The so-called "CDF method" is one way to find the distribution of a
the transformation $Y = g(X)$ of a random variable $X$ with a known CDF.
Let's look at a simpler example first: Suppose $X sim mathsfUniv(0,1)$ and find the CDF of $Y = g(X) = sqrtX.$ The support of $X$ is $(0,1)$ and it is clear that the support of $Y$ will also be $(0,1).$
The CDF of $X$ is $F_X(x) = x,$ for $x in (0,1).$ Then the CDF of $Y$ is
$$P(Y le y) = P(g(X) le y) = P(sqrtX le y) = P(X le y^2) = y^2,$$
for $y in (0,1).$ The last step uses $F_X(x) = P(X le x) = x,$ where $y^2 = x.$ Thus the PDF of $Y$ is $f_Y(y) = F_Y^prime(y) = dy^2/dy = 2y,$ which
we recognize as the PDF of $mathsfBeta(2,1).$
Illustrating this with a random sample of $n = 10^5$ observations $X_i$ from $mathsfUnif(0,1),$ we have the following results (in R):
set.seed(615)
x = runif(10^5, 0, 1); y = sqrt(x)
par(mfrow=c(1,2))
hist(x, prob=T, col="skyblue2", main="X ~ UNIF(0,1)")
curve(dunif(x, 0, 1), add=T, n=10001, lwd=2, col="brown")
hist(y, prob=T, col="skyblue2", main="Y ~ BETA(2,1)")
curve(dbeta(x, 2, 1), add=T, n=10001, lwd=2, col="brown")
par(mfrow=c(1,1))
Your Question. Now let's do a similar procedure for $X$ with CDF $F_X(x) = P(X le x)
= (1 + e^-x)^-theta,$ for $theta > 0$ and the transformation
$Y = g(X) = log(1+e^-X),$ which has support $(0, infty).$
Using the CDF method again, we have:
$$F_Y(y) = P(Yle y) = P(log(1 + e^-X)le y) = P(1+e^-X le e^y)\
=P(e^-X le e^y - 1) = P(-X le log(e^y -1))\ = P(X ge -log(e^y -1)) = cdots,$$
So, $F_y(y) = 1-e^-theta y,$ for $y > 0,$ as claimed.
We illustrate with a random sample of $n = 10^5$ observations from the
original logistic distribution with $theta = 1.$ This distribution can be sampled in terms of standard uniform distributions as shown in the R code;
see Wikipedia, second bullet under Related Distributions.
set.seed(2019)
u = runif(10^5); x = log(u) - log(1-u)
y = log(1 + exp(-x))
par(mfrow=c(1,2))
hist(x, prob=T, br=30, ylim=c(0,.25), col="skyblue2", main="Logistic")
curve(exp(-x)/(1+exp(-x))^2, add=T, lwd=2, col="brown")
hist(y, prob=T, ylim=c(0,1), col="skyblue2", main="Exponential")
curve(dexp(x,1), add=T, lwd=2, n=10001, col="brown")
par(mfrow=c(1,1))
$endgroup$
Introduction. The so-called "CDF method" is one way to find the distribution of a
the transformation $Y = g(X)$ of a random variable $X$ with a known CDF.
Let's look at a simpler example first: Suppose $X sim mathsfUniv(0,1)$ and find the CDF of $Y = g(X) = sqrtX.$ The support of $X$ is $(0,1)$ and it is clear that the support of $Y$ will also be $(0,1).$
The CDF of $X$ is $F_X(x) = x,$ for $x in (0,1).$ Then the CDF of $Y$ is
$$P(Y le y) = P(g(X) le y) = P(sqrtX le y) = P(X le y^2) = y^2,$$
for $y in (0,1).$ The last step uses $F_X(x) = P(X le x) = x,$ where $y^2 = x.$ Thus the PDF of $Y$ is $f_Y(y) = F_Y^prime(y) = dy^2/dy = 2y,$ which
we recognize as the PDF of $mathsfBeta(2,1).$
Illustrating this with a random sample of $n = 10^5$ observations $X_i$ from $mathsfUnif(0,1),$ we have the following results (in R):
set.seed(615)
x = runif(10^5, 0, 1); y = sqrt(x)
par(mfrow=c(1,2))
hist(x, prob=T, col="skyblue2", main="X ~ UNIF(0,1)")
curve(dunif(x, 0, 1), add=T, n=10001, lwd=2, col="brown")
hist(y, prob=T, col="skyblue2", main="Y ~ BETA(2,1)")
curve(dbeta(x, 2, 1), add=T, n=10001, lwd=2, col="brown")
par(mfrow=c(1,1))
Your Question. Now let's do a similar procedure for $X$ with CDF $F_X(x) = P(X le x)
= (1 + e^-x)^-theta,$ for $theta > 0$ and the transformation
$Y = g(X) = log(1+e^-X),$ which has support $(0, infty).$
Using the CDF method again, we have:
$$F_Y(y) = P(Yle y) = P(log(1 + e^-X)le y) = P(1+e^-X le e^y)\
=P(e^-X le e^y - 1) = P(-X le log(e^y -1))\ = P(X ge -log(e^y -1)) = cdots,$$
So, $F_y(y) = 1-e^-theta y,$ for $y > 0,$ as claimed.
We illustrate with a random sample of $n = 10^5$ observations from the
original logistic distribution with $theta = 1.$ This distribution can be sampled in terms of standard uniform distributions as shown in the R code;
see Wikipedia, second bullet under Related Distributions.
set.seed(2019)
u = runif(10^5); x = log(u) - log(1-u)
y = log(1 + exp(-x))
par(mfrow=c(1,2))
hist(x, prob=T, br=30, ylim=c(0,.25), col="skyblue2", main="Logistic")
curve(exp(-x)/(1+exp(-x))^2, add=T, lwd=2, col="brown")
hist(y, prob=T, ylim=c(0,1), col="skyblue2", main="Exponential")
curve(dexp(x,1), add=T, lwd=2, n=10001, col="brown")
par(mfrow=c(1,1))
edited 3 hours ago
answered 5 hours ago
BruceETBruceET
8,9081823
8,9081823
add a comment |
add a comment |
$begingroup$
beginalign
& log(1+e^-X) le y \[12pt]
& 1+e^-X le e^y \
& textsince log text and exp \
& textare increasing functions \[12pt]
& e^-X le e^y - 1 \[12pt]
& -X le log(e^y - 1) \
& textsince log text and exp \
& textare increasing functions \[12pt]
& X ge -log(e^y - 1)
endalign
$endgroup$
add a comment |
$begingroup$
beginalign
& log(1+e^-X) le y \[12pt]
& 1+e^-X le e^y \
& textsince log text and exp \
& textare increasing functions \[12pt]
& e^-X le e^y - 1 \[12pt]
& -X le log(e^y - 1) \
& textsince log text and exp \
& textare increasing functions \[12pt]
& X ge -log(e^y - 1)
endalign
$endgroup$
add a comment |
$begingroup$
beginalign
& log(1+e^-X) le y \[12pt]
& 1+e^-X le e^y \
& textsince log text and exp \
& textare increasing functions \[12pt]
& e^-X le e^y - 1 \[12pt]
& -X le log(e^y - 1) \
& textsince log text and exp \
& textare increasing functions \[12pt]
& X ge -log(e^y - 1)
endalign
$endgroup$
beginalign
& log(1+e^-X) le y \[12pt]
& 1+e^-X le e^y \
& textsince log text and exp \
& textare increasing functions \[12pt]
& e^-X le e^y - 1 \[12pt]
& -X le log(e^y - 1) \
& textsince log text and exp \
& textare increasing functions \[12pt]
& X ge -log(e^y - 1)
endalign
answered 5 hours ago
Michael HardyMichael Hardy
4,4541430
4,4541430
add a comment |
add a comment |
$begingroup$
Question 1:
By definition, FY(y) = Pr(Y<=y)
And because we know that Y=log(1+e^−X)
Then FY(y) = Pr(log(1+e^−X) <= y)
Question 2:
All they're doing there is starting with log(1+e^−X)≤y
and simply solving for X
which entails, first doing e^ on both sides:
1+e^−X ≤ e^y
then sending the 1 to the right:
e^−X ≤ e^y - 1
then doing log on both sides:
−X ≤ log(e^y - 1)
and finally multiplying both sides by -1, which changes the direction of the ≤
So you end up with:
X≥−log(e^y−1)
which is what they got
New contributor
Dave is a new contributor to this site. Take care in asking for clarification, commenting, and answering.
Check out our Code of Conduct.
$endgroup$
add a comment |
$begingroup$
Question 1:
By definition, FY(y) = Pr(Y<=y)
And because we know that Y=log(1+e^−X)
Then FY(y) = Pr(log(1+e^−X) <= y)
Question 2:
All they're doing there is starting with log(1+e^−X)≤y
and simply solving for X
which entails, first doing e^ on both sides:
1+e^−X ≤ e^y
then sending the 1 to the right:
e^−X ≤ e^y - 1
then doing log on both sides:
−X ≤ log(e^y - 1)
and finally multiplying both sides by -1, which changes the direction of the ≤
So you end up with:
X≥−log(e^y−1)
which is what they got
New contributor
Dave is a new contributor to this site. Take care in asking for clarification, commenting, and answering.
Check out our Code of Conduct.
$endgroup$
add a comment |
$begingroup$
Question 1:
By definition, FY(y) = Pr(Y<=y)
And because we know that Y=log(1+e^−X)
Then FY(y) = Pr(log(1+e^−X) <= y)
Question 2:
All they're doing there is starting with log(1+e^−X)≤y
and simply solving for X
which entails, first doing e^ on both sides:
1+e^−X ≤ e^y
then sending the 1 to the right:
e^−X ≤ e^y - 1
then doing log on both sides:
−X ≤ log(e^y - 1)
and finally multiplying both sides by -1, which changes the direction of the ≤
So you end up with:
X≥−log(e^y−1)
which is what they got
New contributor
Dave is a new contributor to this site. Take care in asking for clarification, commenting, and answering.
Check out our Code of Conduct.
$endgroup$
Question 1:
By definition, FY(y) = Pr(Y<=y)
And because we know that Y=log(1+e^−X)
Then FY(y) = Pr(log(1+e^−X) <= y)
Question 2:
All they're doing there is starting with log(1+e^−X)≤y
and simply solving for X
which entails, first doing e^ on both sides:
1+e^−X ≤ e^y
then sending the 1 to the right:
e^−X ≤ e^y - 1
then doing log on both sides:
−X ≤ log(e^y - 1)
and finally multiplying both sides by -1, which changes the direction of the ≤
So you end up with:
X≥−log(e^y−1)
which is what they got
New contributor
Dave is a new contributor to this site. Take care in asking for clarification, commenting, and answering.
Check out our Code of Conduct.
New contributor
Dave is a new contributor to this site. Take care in asking for clarification, commenting, and answering.
Check out our Code of Conduct.
answered 5 hours ago
DaveDave
745
745
New contributor
Dave is a new contributor to this site. Take care in asking for clarification, commenting, and answering.
Check out our Code of Conduct.
New contributor
Dave is a new contributor to this site. Take care in asking for clarification, commenting, and answering.
Check out our Code of Conduct.
add a comment |
add a comment |
Thanks for contributing an answer to Cross Validated!
- Please be sure to answer the question. Provide details and share your research!
But avoid …
- Asking for help, clarification, or responding to other answers.
- Making statements based on opinion; back them up with references or personal experience.
Use MathJax to format equations. MathJax reference.
To learn more, see our tips on writing great answers.
Sign up or log in
StackExchange.ready(function ()
StackExchange.helpers.onClickDraftSave('#login-link');
);
Sign up using Google
Sign up using Facebook
Sign up using Email and Password
Post as a guest
Required, but never shown
StackExchange.ready(
function ()
StackExchange.openid.initPostLogin('.new-post-login', 'https%3a%2f%2fstats.stackexchange.com%2fquestions%2f413187%2fderivation-of-cdf-of-a-function-that-results-in-an-exponential-distribution%23new-answer', 'question_page');
);
Post as a guest
Required, but never shown
Sign up or log in
StackExchange.ready(function ()
StackExchange.helpers.onClickDraftSave('#login-link');
);
Sign up using Google
Sign up using Facebook
Sign up using Email and Password
Post as a guest
Required, but never shown
Sign up or log in
StackExchange.ready(function ()
StackExchange.helpers.onClickDraftSave('#login-link');
);
Sign up using Google
Sign up using Facebook
Sign up using Email and Password
Post as a guest
Required, but never shown
Sign up or log in
StackExchange.ready(function ()
StackExchange.helpers.onClickDraftSave('#login-link');
);
Sign up using Google
Sign up using Facebook
Sign up using Email and Password
Sign up using Google
Sign up using Facebook
Sign up using Email and Password
Post as a guest
Required, but never shown
Required, but never shown
Required, but never shown
Required, but never shown
Required, but never shown
Required, but never shown
Required, but never shown
Required, but never shown
Required, but never shown
jVFuogiZYJAi 2
$begingroup$
Hint: By definition, for each real number $alpha$, the value of tthe CDF $F_Y$ at $alpha$, usually expressed as $F_Y(alpha)$, equals the probability that $Y$ is smaller than $alpha$. So, knowing this, can you figure out for yourself why the implication $$Y = log(1 + e^-X) Longrightarrow F_Y(y) = Pr(Y leq y) = Pr( log(1+e^-X) le y )$$ holds??
$endgroup$
– Dilip Sarwate
5 hours ago
$begingroup$
In what I wrote above, for "$Y$ is smaller than $alpha$", please substitute "$Y$ is no larger than $alpha$".
$endgroup$
– Dilip Sarwate
5 hours ago