If p-value is exactly 1 (1.0000000), what are the confidence interval limits?If we fail to reject the null hypothesis in a large study, isn't it evidence for the null?Relation between confidence interval and testing statistical hypothesis for t-testConfidence interval for values for a fitted lineWhat should I do when a confidence interval includes an impossible range of values?Confidence Interval for Linear RegressionWhy does Fisher's exact test produce the same p-value regardless of my predictor variable?Estimated Mean and Confidence Interval and Predicted Value and Prediction IntervalUpper & lower bound of confidence interval of mean
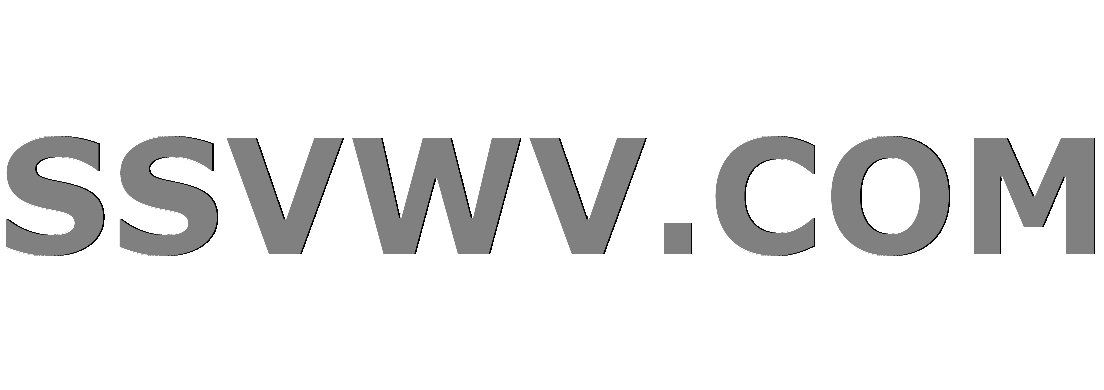
Multi tool use
My boss says "This will help us better view the utilization of your services." Does this mean my job is ending in this organisation?
In-universe, why does Doc Brown program the time machine to go to 1955?
How do I stop making people jump at home and at work?
stackrel, vertical alignment and math operators
Informed Consent Research Question
Why is k-means used for non normally distributed data?
Disney Musicians Ordering
Importance of electrolytic capacitor size
Divide Numbers by 0
Punishment in pacifist society
Which is the best password hashing algorithm in .NET Core?
How does Harry wear the invisibility cloak?
Does the Scrying spell require you to have a clear path to the target in order to work?
Do we know the problems the University of Manchester's Transistor Computer was intended to solve?
A question about dihedral group
Remove ads in Viber for PC
Why do old games use flashing as means of showing damage?
Why don't they build airplanes from 3D printer plastic?
What is the converted mana cost of land cards?
How can I design a magically-induced coma?
Solve this icositetragram
How to disambiguate between various meditation practices?
To which country did MiGs in Top Gun belong?
How did Gollum know Sauron was gathering the Haradrim to make war?
If p-value is exactly 1 (1.0000000), what are the confidence interval limits?
If we fail to reject the null hypothesis in a large study, isn't it evidence for the null?Relation between confidence interval and testing statistical hypothesis for t-testConfidence interval for values for a fitted lineWhat should I do when a confidence interval includes an impossible range of values?Confidence Interval for Linear RegressionWhy does Fisher's exact test produce the same p-value regardless of my predictor variable?Estimated Mean and Confidence Interval and Predicted Value and Prediction IntervalUpper & lower bound of confidence interval of mean
.everyoneloves__top-leaderboard:empty,.everyoneloves__mid-leaderboard:empty,.everyoneloves__bot-mid-leaderboard:empty margin-bottom:0;
$begingroup$
This is purely a hypothetical question. A very common statement is that H0 is never true, it´s just a matter of sample size.
Let´s assume that for real there is absolutely no measurable difference between two means (u0=u1) drawn from normally distributed population (for both u=0 and SD estimated=1). We assume N=16 per group and we use t-test. This would mean that p-value is 1.00000 indicating that there is absolutely no discrepancy from H0. This would indicate that test statistic is 0. Mean difference between groups would be 0. What would be the limits of 95% confidence interval for the mean difference in this case? Would they be [0.0,0.0]?
EDIT: Main point in my question was that when can we really say that H0 is true, ie. u1=U2 in this case?
confidence-interval p-value
$endgroup$
|
show 1 more comment
$begingroup$
This is purely a hypothetical question. A very common statement is that H0 is never true, it´s just a matter of sample size.
Let´s assume that for real there is absolutely no measurable difference between two means (u0=u1) drawn from normally distributed population (for both u=0 and SD estimated=1). We assume N=16 per group and we use t-test. This would mean that p-value is 1.00000 indicating that there is absolutely no discrepancy from H0. This would indicate that test statistic is 0. Mean difference between groups would be 0. What would be the limits of 95% confidence interval for the mean difference in this case? Would they be [0.0,0.0]?
EDIT: Main point in my question was that when can we really say that H0 is true, ie. u1=U2 in this case?
confidence-interval p-value
$endgroup$
$begingroup$
I'd say that this was already answered in here stats.stackexchange.com/questions/275677/…, but I'm not insisting on it.
$endgroup$
– Tim♦
8 hours ago
$begingroup$
If p was exactly unity, then it would be exactly 100% risky to reject the null hypothesis, whatever it was. So whatever lead to p = 1 would have given no reason whatsoever to doubt the null hypothesis.
$endgroup$
– Ed V
8 hours ago
$begingroup$
I’m having trouble coming up with a way to get $p=1$ with positive population variances.
$endgroup$
– Dave
8 hours ago
$begingroup$
I'm no expert, but my understanding of the logic of hypothesis testing is that $pRightarrow q$, where $p$ is some condition on the p-value and $q$ is rejecting the null hypothesis. You CANNOT conclude that $sim pRightarrow sim q$, No matter how confident you are about $sim p$, which I guess answers your question.
$endgroup$
– idnavid
8 hours ago
$begingroup$
The CI will be symmetric around $0$ but it won't be zero because we would still have a finite sample effect. As $n$ approach infinity we would get the CI becoming narrower and narrower but that is not due to the difference being zero. Note that the "narrowing" of the CI would be true even if $mu_A neq mu_B$.
$endgroup$
– usεr11852
7 hours ago
|
show 1 more comment
$begingroup$
This is purely a hypothetical question. A very common statement is that H0 is never true, it´s just a matter of sample size.
Let´s assume that for real there is absolutely no measurable difference between two means (u0=u1) drawn from normally distributed population (for both u=0 and SD estimated=1). We assume N=16 per group and we use t-test. This would mean that p-value is 1.00000 indicating that there is absolutely no discrepancy from H0. This would indicate that test statistic is 0. Mean difference between groups would be 0. What would be the limits of 95% confidence interval for the mean difference in this case? Would they be [0.0,0.0]?
EDIT: Main point in my question was that when can we really say that H0 is true, ie. u1=U2 in this case?
confidence-interval p-value
$endgroup$
This is purely a hypothetical question. A very common statement is that H0 is never true, it´s just a matter of sample size.
Let´s assume that for real there is absolutely no measurable difference between two means (u0=u1) drawn from normally distributed population (for both u=0 and SD estimated=1). We assume N=16 per group and we use t-test. This would mean that p-value is 1.00000 indicating that there is absolutely no discrepancy from H0. This would indicate that test statistic is 0. Mean difference between groups would be 0. What would be the limits of 95% confidence interval for the mean difference in this case? Would they be [0.0,0.0]?
EDIT: Main point in my question was that when can we really say that H0 is true, ie. u1=U2 in this case?
confidence-interval p-value
confidence-interval p-value
edited 31 mins ago
arkiaamu
asked 9 hours ago
arkiaamuarkiaamu
2702 silver badges9 bronze badges
2702 silver badges9 bronze badges
$begingroup$
I'd say that this was already answered in here stats.stackexchange.com/questions/275677/…, but I'm not insisting on it.
$endgroup$
– Tim♦
8 hours ago
$begingroup$
If p was exactly unity, then it would be exactly 100% risky to reject the null hypothesis, whatever it was. So whatever lead to p = 1 would have given no reason whatsoever to doubt the null hypothesis.
$endgroup$
– Ed V
8 hours ago
$begingroup$
I’m having trouble coming up with a way to get $p=1$ with positive population variances.
$endgroup$
– Dave
8 hours ago
$begingroup$
I'm no expert, but my understanding of the logic of hypothesis testing is that $pRightarrow q$, where $p$ is some condition on the p-value and $q$ is rejecting the null hypothesis. You CANNOT conclude that $sim pRightarrow sim q$, No matter how confident you are about $sim p$, which I guess answers your question.
$endgroup$
– idnavid
8 hours ago
$begingroup$
The CI will be symmetric around $0$ but it won't be zero because we would still have a finite sample effect. As $n$ approach infinity we would get the CI becoming narrower and narrower but that is not due to the difference being zero. Note that the "narrowing" of the CI would be true even if $mu_A neq mu_B$.
$endgroup$
– usεr11852
7 hours ago
|
show 1 more comment
$begingroup$
I'd say that this was already answered in here stats.stackexchange.com/questions/275677/…, but I'm not insisting on it.
$endgroup$
– Tim♦
8 hours ago
$begingroup$
If p was exactly unity, then it would be exactly 100% risky to reject the null hypothesis, whatever it was. So whatever lead to p = 1 would have given no reason whatsoever to doubt the null hypothesis.
$endgroup$
– Ed V
8 hours ago
$begingroup$
I’m having trouble coming up with a way to get $p=1$ with positive population variances.
$endgroup$
– Dave
8 hours ago
$begingroup$
I'm no expert, but my understanding of the logic of hypothesis testing is that $pRightarrow q$, where $p$ is some condition on the p-value and $q$ is rejecting the null hypothesis. You CANNOT conclude that $sim pRightarrow sim q$, No matter how confident you are about $sim p$, which I guess answers your question.
$endgroup$
– idnavid
8 hours ago
$begingroup$
The CI will be symmetric around $0$ but it won't be zero because we would still have a finite sample effect. As $n$ approach infinity we would get the CI becoming narrower and narrower but that is not due to the difference being zero. Note that the "narrowing" of the CI would be true even if $mu_A neq mu_B$.
$endgroup$
– usεr11852
7 hours ago
$begingroup$
I'd say that this was already answered in here stats.stackexchange.com/questions/275677/…, but I'm not insisting on it.
$endgroup$
– Tim♦
8 hours ago
$begingroup$
I'd say that this was already answered in here stats.stackexchange.com/questions/275677/…, but I'm not insisting on it.
$endgroup$
– Tim♦
8 hours ago
$begingroup$
If p was exactly unity, then it would be exactly 100% risky to reject the null hypothesis, whatever it was. So whatever lead to p = 1 would have given no reason whatsoever to doubt the null hypothesis.
$endgroup$
– Ed V
8 hours ago
$begingroup$
If p was exactly unity, then it would be exactly 100% risky to reject the null hypothesis, whatever it was. So whatever lead to p = 1 would have given no reason whatsoever to doubt the null hypothesis.
$endgroup$
– Ed V
8 hours ago
$begingroup$
I’m having trouble coming up with a way to get $p=1$ with positive population variances.
$endgroup$
– Dave
8 hours ago
$begingroup$
I’m having trouble coming up with a way to get $p=1$ with positive population variances.
$endgroup$
– Dave
8 hours ago
$begingroup$
I'm no expert, but my understanding of the logic of hypothesis testing is that $pRightarrow q$, where $p$ is some condition on the p-value and $q$ is rejecting the null hypothesis. You CANNOT conclude that $sim pRightarrow sim q$, No matter how confident you are about $sim p$, which I guess answers your question.
$endgroup$
– idnavid
8 hours ago
$begingroup$
I'm no expert, but my understanding of the logic of hypothesis testing is that $pRightarrow q$, where $p$ is some condition on the p-value and $q$ is rejecting the null hypothesis. You CANNOT conclude that $sim pRightarrow sim q$, No matter how confident you are about $sim p$, which I guess answers your question.
$endgroup$
– idnavid
8 hours ago
$begingroup$
The CI will be symmetric around $0$ but it won't be zero because we would still have a finite sample effect. As $n$ approach infinity we would get the CI becoming narrower and narrower but that is not due to the difference being zero. Note that the "narrowing" of the CI would be true even if $mu_A neq mu_B$.
$endgroup$
– usεr11852
7 hours ago
$begingroup$
The CI will be symmetric around $0$ but it won't be zero because we would still have a finite sample effect. As $n$ approach infinity we would get the CI becoming narrower and narrower but that is not due to the difference being zero. Note that the "narrowing" of the CI would be true even if $mu_A neq mu_B$.
$endgroup$
– usεr11852
7 hours ago
|
show 1 more comment
4 Answers
4
active
oldest
votes
$begingroup$
A confidence interval for a t-test is of the form $barx_1 - barx_2 pm t_textcrit, alphas_barx_1 - barx_2$, where $barx_1$ and $barx_2$ are the sample means, $t_textcrit, alpha$ is the critical $t$ value at the given $alpha$, and $s_barx_1 - barx_2$ is the standard error of the difference in means. If $p=1.0$, then $barx_1 - barx_2 =0$. So the formula is just $pm t_textcrit, alphas_barx_1 - barx_2$, and the limits are just $-t_textcrit, alphas_barx_1 - barx_2$, $t_textcrit, alphas_barx_1 - barx_2$.
I'm not sure why you would think the limits would be $0,0.$ The critical $t$ value is not zero and the standard error of the mean difference is not zero.
$endgroup$
add a comment |
$begingroup$
Being super-lazy, using R to solve the problem numerically rather than doing the calculations by hand:
Define a function that will give normally distributed values with a mean of (almost!) exactly zero and a SD of exactly 1:
rn2 <- function(n) r <- rnorm(n); c(scale(r))
Run a t-test:
t.test(rn2(16),rn2(16))
Welch Two Sample t-test
data: rn2(16) and rn2(16)
t = 1.7173e-17, df = 30, p-value = 1
alternative hypothesis: true difference in means is not equal to 0
95 percent confidence interval:
-0.7220524 0.7220524
sample estimates:
mean of x mean of y
6.938894e-18 8.673617e-19
The means are not exactly zero because of floating-point imprecision.
$endgroup$
add a comment |
$begingroup$
Your question is somewhat underdefined. There are lots of tests. Chances are you refer to the two-sample t-test. It makes a difference whether the sd is known or estimated (Gauss test vs. t-test). N=16+16 means N=16 in each of two samples? Or did you want to have a really large sample size such as N=16e+16?
In any case, nothing stops you from using standard t- or Gauss-formulae for computing the confidence interval - all informations needed are given in your question (assuming that you know exactly what you mean with your notation even though I don't). p=1 doesn't mean that there's anything wrong with that. Note that p=1 does not mean that you can be particularly sure that the H0 is true. Random variation is still present and if u0=u1 can happen under the H0, it can also happen if the true value of u0 is slightly different from the true u1, so there will be more in the confidence interval than just equality.
$endgroup$
$begingroup$
I did some editing, I hope it's more defined now.
$endgroup$
– arkiaamu
8 hours ago
add a comment |
$begingroup$
It is difficult to have a cogent philosophical discussion about things
that have 0 probability of happening. So I will show you some examples
that relate to your question.
If you have two enormous independent samples from the same distribution,
then both samples will still have some variability, the pooled 2-sample t statistic will
be near, but not exactly 0, the P-value will be distributed as
$mathsfUnif(0,1),$ and the 95% confidence interval will be very short
and centered very near $0.$
An example of one such dataset and t test:
set.seed(902)
x1 = rnorm(10^5, 100, 15)
x2 = rnorm(10^5, 100, 15)
t.test(x1, x2, var.eq=T)
Two Sample t-test
data: x1 and x2
t = -0.41372, df = 2e+05, p-value = 0.6791
alternative hypothesis: true difference in means is not equal to 0
95 percent confidence interval:
-0.1591659 0.1036827
sample estimates:
mean of x mean of y
99.96403 99.99177
Here are summarized results from 10,000 such situations. First, the distribution of P-values.
set.seed(2019)
pv = replicate(10^4,
t.test(rnorm(10^5,100,15),rnorm(10^5,100,15),var.eq=T)$p.val)
mean(pv)
[1] 0.5007066 # aprx 1/2
hist(pv, prob=T, col="skyblue2", main="Simulated P-values")
curve(dunif(x), add=T, col="red", lwd=2, n=10001)
Next the test statistic:
set.seed(2019) # same seed as above, so same 10^4 datasets
st = replicate(10^4,
t.test(rnorm(10^5,100,15),rnorm(10^5,100,15),var.eq=T)$stat)
mean(st)
[1] 0.002810332 # aprx 0
hist(st, prob=T, col="skyblue2", main="Simulated P-values")
curve(dt(x, df=2e+05), add=T, col="red", lwd=2, n=10001)
And so on for the width of the CI.
set.seed(2019)
w.ci = replicate(10^4,
diff(t.test(rnorm(10^5,100,15),
rnorm(10^5,100,15),var.eq=T)$conf.int))
mean(w.ci)
[1] 0.2629603
It is almost impossible to get a
P-value of unity doing an exact test with continuous data, where
assumptions are met. So much so, that a wise statistician will ponder
what might have gone wrong upon seeing a P-value of 1.
For example, you might give the software two identical large samples.
The programming will carry on as if these are two independent samples, and
give strange results. But even then the CI will not be of 0 width.
set.seed(902)
x1 = rnorm(10^5, 100, 15)
x2 = x1
t.test(x1, x2, var.eq=T)
Two Sample t-test
data: x1 and x2
t = 0, df = 2e+05, p-value = 1
alternative hypothesis: true difference in means is not equal to 0
95 percent confidence interval:
-0.1316593 0.1316593
sample estimates:
mean of x mean of y
99.96403 99.96403
$endgroup$
add a comment |
Your Answer
StackExchange.ready(function()
var channelOptions =
tags: "".split(" "),
id: "65"
;
initTagRenderer("".split(" "), "".split(" "), channelOptions);
StackExchange.using("externalEditor", function()
// Have to fire editor after snippets, if snippets enabled
if (StackExchange.settings.snippets.snippetsEnabled)
StackExchange.using("snippets", function()
createEditor();
);
else
createEditor();
);
function createEditor()
StackExchange.prepareEditor(
heartbeatType: 'answer',
autoActivateHeartbeat: false,
convertImagesToLinks: false,
noModals: true,
showLowRepImageUploadWarning: true,
reputationToPostImages: null,
bindNavPrevention: true,
postfix: "",
imageUploader:
brandingHtml: "Powered by u003ca class="icon-imgur-white" href="https://imgur.com/"u003eu003c/au003e",
contentPolicyHtml: "User contributions licensed under u003ca href="https://creativecommons.org/licenses/by-sa/3.0/"u003ecc by-sa 3.0 with attribution requiredu003c/au003e u003ca href="https://stackoverflow.com/legal/content-policy"u003e(content policy)u003c/au003e",
allowUrls: true
,
onDemand: true,
discardSelector: ".discard-answer"
,immediatelyShowMarkdownHelp:true
);
);
Sign up or log in
StackExchange.ready(function ()
StackExchange.helpers.onClickDraftSave('#login-link');
);
Sign up using Google
Sign up using Facebook
Sign up using Email and Password
Post as a guest
Required, but never shown
StackExchange.ready(
function ()
StackExchange.openid.initPostLogin('.new-post-login', 'https%3a%2f%2fstats.stackexchange.com%2fquestions%2f424656%2fif-p-value-is-exactly-1-1-0000000-what-are-the-confidence-interval-limits%23new-answer', 'question_page');
);
Post as a guest
Required, but never shown
4 Answers
4
active
oldest
votes
4 Answers
4
active
oldest
votes
active
oldest
votes
active
oldest
votes
$begingroup$
A confidence interval for a t-test is of the form $barx_1 - barx_2 pm t_textcrit, alphas_barx_1 - barx_2$, where $barx_1$ and $barx_2$ are the sample means, $t_textcrit, alpha$ is the critical $t$ value at the given $alpha$, and $s_barx_1 - barx_2$ is the standard error of the difference in means. If $p=1.0$, then $barx_1 - barx_2 =0$. So the formula is just $pm t_textcrit, alphas_barx_1 - barx_2$, and the limits are just $-t_textcrit, alphas_barx_1 - barx_2$, $t_textcrit, alphas_barx_1 - barx_2$.
I'm not sure why you would think the limits would be $0,0.$ The critical $t$ value is not zero and the standard error of the mean difference is not zero.
$endgroup$
add a comment |
$begingroup$
A confidence interval for a t-test is of the form $barx_1 - barx_2 pm t_textcrit, alphas_barx_1 - barx_2$, where $barx_1$ and $barx_2$ are the sample means, $t_textcrit, alpha$ is the critical $t$ value at the given $alpha$, and $s_barx_1 - barx_2$ is the standard error of the difference in means. If $p=1.0$, then $barx_1 - barx_2 =0$. So the formula is just $pm t_textcrit, alphas_barx_1 - barx_2$, and the limits are just $-t_textcrit, alphas_barx_1 - barx_2$, $t_textcrit, alphas_barx_1 - barx_2$.
I'm not sure why you would think the limits would be $0,0.$ The critical $t$ value is not zero and the standard error of the mean difference is not zero.
$endgroup$
add a comment |
$begingroup$
A confidence interval for a t-test is of the form $barx_1 - barx_2 pm t_textcrit, alphas_barx_1 - barx_2$, where $barx_1$ and $barx_2$ are the sample means, $t_textcrit, alpha$ is the critical $t$ value at the given $alpha$, and $s_barx_1 - barx_2$ is the standard error of the difference in means. If $p=1.0$, then $barx_1 - barx_2 =0$. So the formula is just $pm t_textcrit, alphas_barx_1 - barx_2$, and the limits are just $-t_textcrit, alphas_barx_1 - barx_2$, $t_textcrit, alphas_barx_1 - barx_2$.
I'm not sure why you would think the limits would be $0,0.$ The critical $t$ value is not zero and the standard error of the mean difference is not zero.
$endgroup$
A confidence interval for a t-test is of the form $barx_1 - barx_2 pm t_textcrit, alphas_barx_1 - barx_2$, where $barx_1$ and $barx_2$ are the sample means, $t_textcrit, alpha$ is the critical $t$ value at the given $alpha$, and $s_barx_1 - barx_2$ is the standard error of the difference in means. If $p=1.0$, then $barx_1 - barx_2 =0$. So the formula is just $pm t_textcrit, alphas_barx_1 - barx_2$, and the limits are just $-t_textcrit, alphas_barx_1 - barx_2$, $t_textcrit, alphas_barx_1 - barx_2$.
I'm not sure why you would think the limits would be $0,0.$ The critical $t$ value is not zero and the standard error of the mean difference is not zero.
edited 2 hours ago
Michael Hardy
4,74415 silver badges30 bronze badges
4,74415 silver badges30 bronze badges
answered 6 hours ago
NoahNoah
6,0821 gold badge5 silver badges21 bronze badges
6,0821 gold badge5 silver badges21 bronze badges
add a comment |
add a comment |
$begingroup$
Being super-lazy, using R to solve the problem numerically rather than doing the calculations by hand:
Define a function that will give normally distributed values with a mean of (almost!) exactly zero and a SD of exactly 1:
rn2 <- function(n) r <- rnorm(n); c(scale(r))
Run a t-test:
t.test(rn2(16),rn2(16))
Welch Two Sample t-test
data: rn2(16) and rn2(16)
t = 1.7173e-17, df = 30, p-value = 1
alternative hypothesis: true difference in means is not equal to 0
95 percent confidence interval:
-0.7220524 0.7220524
sample estimates:
mean of x mean of y
6.938894e-18 8.673617e-19
The means are not exactly zero because of floating-point imprecision.
$endgroup$
add a comment |
$begingroup$
Being super-lazy, using R to solve the problem numerically rather than doing the calculations by hand:
Define a function that will give normally distributed values with a mean of (almost!) exactly zero and a SD of exactly 1:
rn2 <- function(n) r <- rnorm(n); c(scale(r))
Run a t-test:
t.test(rn2(16),rn2(16))
Welch Two Sample t-test
data: rn2(16) and rn2(16)
t = 1.7173e-17, df = 30, p-value = 1
alternative hypothesis: true difference in means is not equal to 0
95 percent confidence interval:
-0.7220524 0.7220524
sample estimates:
mean of x mean of y
6.938894e-18 8.673617e-19
The means are not exactly zero because of floating-point imprecision.
$endgroup$
add a comment |
$begingroup$
Being super-lazy, using R to solve the problem numerically rather than doing the calculations by hand:
Define a function that will give normally distributed values with a mean of (almost!) exactly zero and a SD of exactly 1:
rn2 <- function(n) r <- rnorm(n); c(scale(r))
Run a t-test:
t.test(rn2(16),rn2(16))
Welch Two Sample t-test
data: rn2(16) and rn2(16)
t = 1.7173e-17, df = 30, p-value = 1
alternative hypothesis: true difference in means is not equal to 0
95 percent confidence interval:
-0.7220524 0.7220524
sample estimates:
mean of x mean of y
6.938894e-18 8.673617e-19
The means are not exactly zero because of floating-point imprecision.
$endgroup$
Being super-lazy, using R to solve the problem numerically rather than doing the calculations by hand:
Define a function that will give normally distributed values with a mean of (almost!) exactly zero and a SD of exactly 1:
rn2 <- function(n) r <- rnorm(n); c(scale(r))
Run a t-test:
t.test(rn2(16),rn2(16))
Welch Two Sample t-test
data: rn2(16) and rn2(16)
t = 1.7173e-17, df = 30, p-value = 1
alternative hypothesis: true difference in means is not equal to 0
95 percent confidence interval:
-0.7220524 0.7220524
sample estimates:
mean of x mean of y
6.938894e-18 8.673617e-19
The means are not exactly zero because of floating-point imprecision.
answered 4 hours ago
Ben BolkerBen Bolker
25.7k2 gold badges70 silver badges96 bronze badges
25.7k2 gold badges70 silver badges96 bronze badges
add a comment |
add a comment |
$begingroup$
Your question is somewhat underdefined. There are lots of tests. Chances are you refer to the two-sample t-test. It makes a difference whether the sd is known or estimated (Gauss test vs. t-test). N=16+16 means N=16 in each of two samples? Or did you want to have a really large sample size such as N=16e+16?
In any case, nothing stops you from using standard t- or Gauss-formulae for computing the confidence interval - all informations needed are given in your question (assuming that you know exactly what you mean with your notation even though I don't). p=1 doesn't mean that there's anything wrong with that. Note that p=1 does not mean that you can be particularly sure that the H0 is true. Random variation is still present and if u0=u1 can happen under the H0, it can also happen if the true value of u0 is slightly different from the true u1, so there will be more in the confidence interval than just equality.
$endgroup$
$begingroup$
I did some editing, I hope it's more defined now.
$endgroup$
– arkiaamu
8 hours ago
add a comment |
$begingroup$
Your question is somewhat underdefined. There are lots of tests. Chances are you refer to the two-sample t-test. It makes a difference whether the sd is known or estimated (Gauss test vs. t-test). N=16+16 means N=16 in each of two samples? Or did you want to have a really large sample size such as N=16e+16?
In any case, nothing stops you from using standard t- or Gauss-formulae for computing the confidence interval - all informations needed are given in your question (assuming that you know exactly what you mean with your notation even though I don't). p=1 doesn't mean that there's anything wrong with that. Note that p=1 does not mean that you can be particularly sure that the H0 is true. Random variation is still present and if u0=u1 can happen under the H0, it can also happen if the true value of u0 is slightly different from the true u1, so there will be more in the confidence interval than just equality.
$endgroup$
$begingroup$
I did some editing, I hope it's more defined now.
$endgroup$
– arkiaamu
8 hours ago
add a comment |
$begingroup$
Your question is somewhat underdefined. There are lots of tests. Chances are you refer to the two-sample t-test. It makes a difference whether the sd is known or estimated (Gauss test vs. t-test). N=16+16 means N=16 in each of two samples? Or did you want to have a really large sample size such as N=16e+16?
In any case, nothing stops you from using standard t- or Gauss-formulae for computing the confidence interval - all informations needed are given in your question (assuming that you know exactly what you mean with your notation even though I don't). p=1 doesn't mean that there's anything wrong with that. Note that p=1 does not mean that you can be particularly sure that the H0 is true. Random variation is still present and if u0=u1 can happen under the H0, it can also happen if the true value of u0 is slightly different from the true u1, so there will be more in the confidence interval than just equality.
$endgroup$
Your question is somewhat underdefined. There are lots of tests. Chances are you refer to the two-sample t-test. It makes a difference whether the sd is known or estimated (Gauss test vs. t-test). N=16+16 means N=16 in each of two samples? Or did you want to have a really large sample size such as N=16e+16?
In any case, nothing stops you from using standard t- or Gauss-formulae for computing the confidence interval - all informations needed are given in your question (assuming that you know exactly what you mean with your notation even though I don't). p=1 doesn't mean that there's anything wrong with that. Note that p=1 does not mean that you can be particularly sure that the H0 is true. Random variation is still present and if u0=u1 can happen under the H0, it can also happen if the true value of u0 is slightly different from the true u1, so there will be more in the confidence interval than just equality.
answered 8 hours ago
LewianLewian
4546 bronze badges
4546 bronze badges
$begingroup$
I did some editing, I hope it's more defined now.
$endgroup$
– arkiaamu
8 hours ago
add a comment |
$begingroup$
I did some editing, I hope it's more defined now.
$endgroup$
– arkiaamu
8 hours ago
$begingroup$
I did some editing, I hope it's more defined now.
$endgroup$
– arkiaamu
8 hours ago
$begingroup$
I did some editing, I hope it's more defined now.
$endgroup$
– arkiaamu
8 hours ago
add a comment |
$begingroup$
It is difficult to have a cogent philosophical discussion about things
that have 0 probability of happening. So I will show you some examples
that relate to your question.
If you have two enormous independent samples from the same distribution,
then both samples will still have some variability, the pooled 2-sample t statistic will
be near, but not exactly 0, the P-value will be distributed as
$mathsfUnif(0,1),$ and the 95% confidence interval will be very short
and centered very near $0.$
An example of one such dataset and t test:
set.seed(902)
x1 = rnorm(10^5, 100, 15)
x2 = rnorm(10^5, 100, 15)
t.test(x1, x2, var.eq=T)
Two Sample t-test
data: x1 and x2
t = -0.41372, df = 2e+05, p-value = 0.6791
alternative hypothesis: true difference in means is not equal to 0
95 percent confidence interval:
-0.1591659 0.1036827
sample estimates:
mean of x mean of y
99.96403 99.99177
Here are summarized results from 10,000 such situations. First, the distribution of P-values.
set.seed(2019)
pv = replicate(10^4,
t.test(rnorm(10^5,100,15),rnorm(10^5,100,15),var.eq=T)$p.val)
mean(pv)
[1] 0.5007066 # aprx 1/2
hist(pv, prob=T, col="skyblue2", main="Simulated P-values")
curve(dunif(x), add=T, col="red", lwd=2, n=10001)
Next the test statistic:
set.seed(2019) # same seed as above, so same 10^4 datasets
st = replicate(10^4,
t.test(rnorm(10^5,100,15),rnorm(10^5,100,15),var.eq=T)$stat)
mean(st)
[1] 0.002810332 # aprx 0
hist(st, prob=T, col="skyblue2", main="Simulated P-values")
curve(dt(x, df=2e+05), add=T, col="red", lwd=2, n=10001)
And so on for the width of the CI.
set.seed(2019)
w.ci = replicate(10^4,
diff(t.test(rnorm(10^5,100,15),
rnorm(10^5,100,15),var.eq=T)$conf.int))
mean(w.ci)
[1] 0.2629603
It is almost impossible to get a
P-value of unity doing an exact test with continuous data, where
assumptions are met. So much so, that a wise statistician will ponder
what might have gone wrong upon seeing a P-value of 1.
For example, you might give the software two identical large samples.
The programming will carry on as if these are two independent samples, and
give strange results. But even then the CI will not be of 0 width.
set.seed(902)
x1 = rnorm(10^5, 100, 15)
x2 = x1
t.test(x1, x2, var.eq=T)
Two Sample t-test
data: x1 and x2
t = 0, df = 2e+05, p-value = 1
alternative hypothesis: true difference in means is not equal to 0
95 percent confidence interval:
-0.1316593 0.1316593
sample estimates:
mean of x mean of y
99.96403 99.96403
$endgroup$
add a comment |
$begingroup$
It is difficult to have a cogent philosophical discussion about things
that have 0 probability of happening. So I will show you some examples
that relate to your question.
If you have two enormous independent samples from the same distribution,
then both samples will still have some variability, the pooled 2-sample t statistic will
be near, but not exactly 0, the P-value will be distributed as
$mathsfUnif(0,1),$ and the 95% confidence interval will be very short
and centered very near $0.$
An example of one such dataset and t test:
set.seed(902)
x1 = rnorm(10^5, 100, 15)
x2 = rnorm(10^5, 100, 15)
t.test(x1, x2, var.eq=T)
Two Sample t-test
data: x1 and x2
t = -0.41372, df = 2e+05, p-value = 0.6791
alternative hypothesis: true difference in means is not equal to 0
95 percent confidence interval:
-0.1591659 0.1036827
sample estimates:
mean of x mean of y
99.96403 99.99177
Here are summarized results from 10,000 such situations. First, the distribution of P-values.
set.seed(2019)
pv = replicate(10^4,
t.test(rnorm(10^5,100,15),rnorm(10^5,100,15),var.eq=T)$p.val)
mean(pv)
[1] 0.5007066 # aprx 1/2
hist(pv, prob=T, col="skyblue2", main="Simulated P-values")
curve(dunif(x), add=T, col="red", lwd=2, n=10001)
Next the test statistic:
set.seed(2019) # same seed as above, so same 10^4 datasets
st = replicate(10^4,
t.test(rnorm(10^5,100,15),rnorm(10^5,100,15),var.eq=T)$stat)
mean(st)
[1] 0.002810332 # aprx 0
hist(st, prob=T, col="skyblue2", main="Simulated P-values")
curve(dt(x, df=2e+05), add=T, col="red", lwd=2, n=10001)
And so on for the width of the CI.
set.seed(2019)
w.ci = replicate(10^4,
diff(t.test(rnorm(10^5,100,15),
rnorm(10^5,100,15),var.eq=T)$conf.int))
mean(w.ci)
[1] 0.2629603
It is almost impossible to get a
P-value of unity doing an exact test with continuous data, where
assumptions are met. So much so, that a wise statistician will ponder
what might have gone wrong upon seeing a P-value of 1.
For example, you might give the software two identical large samples.
The programming will carry on as if these are two independent samples, and
give strange results. But even then the CI will not be of 0 width.
set.seed(902)
x1 = rnorm(10^5, 100, 15)
x2 = x1
t.test(x1, x2, var.eq=T)
Two Sample t-test
data: x1 and x2
t = 0, df = 2e+05, p-value = 1
alternative hypothesis: true difference in means is not equal to 0
95 percent confidence interval:
-0.1316593 0.1316593
sample estimates:
mean of x mean of y
99.96403 99.96403
$endgroup$
add a comment |
$begingroup$
It is difficult to have a cogent philosophical discussion about things
that have 0 probability of happening. So I will show you some examples
that relate to your question.
If you have two enormous independent samples from the same distribution,
then both samples will still have some variability, the pooled 2-sample t statistic will
be near, but not exactly 0, the P-value will be distributed as
$mathsfUnif(0,1),$ and the 95% confidence interval will be very short
and centered very near $0.$
An example of one such dataset and t test:
set.seed(902)
x1 = rnorm(10^5, 100, 15)
x2 = rnorm(10^5, 100, 15)
t.test(x1, x2, var.eq=T)
Two Sample t-test
data: x1 and x2
t = -0.41372, df = 2e+05, p-value = 0.6791
alternative hypothesis: true difference in means is not equal to 0
95 percent confidence interval:
-0.1591659 0.1036827
sample estimates:
mean of x mean of y
99.96403 99.99177
Here are summarized results from 10,000 such situations. First, the distribution of P-values.
set.seed(2019)
pv = replicate(10^4,
t.test(rnorm(10^5,100,15),rnorm(10^5,100,15),var.eq=T)$p.val)
mean(pv)
[1] 0.5007066 # aprx 1/2
hist(pv, prob=T, col="skyblue2", main="Simulated P-values")
curve(dunif(x), add=T, col="red", lwd=2, n=10001)
Next the test statistic:
set.seed(2019) # same seed as above, so same 10^4 datasets
st = replicate(10^4,
t.test(rnorm(10^5,100,15),rnorm(10^5,100,15),var.eq=T)$stat)
mean(st)
[1] 0.002810332 # aprx 0
hist(st, prob=T, col="skyblue2", main="Simulated P-values")
curve(dt(x, df=2e+05), add=T, col="red", lwd=2, n=10001)
And so on for the width of the CI.
set.seed(2019)
w.ci = replicate(10^4,
diff(t.test(rnorm(10^5,100,15),
rnorm(10^5,100,15),var.eq=T)$conf.int))
mean(w.ci)
[1] 0.2629603
It is almost impossible to get a
P-value of unity doing an exact test with continuous data, where
assumptions are met. So much so, that a wise statistician will ponder
what might have gone wrong upon seeing a P-value of 1.
For example, you might give the software two identical large samples.
The programming will carry on as if these are two independent samples, and
give strange results. But even then the CI will not be of 0 width.
set.seed(902)
x1 = rnorm(10^5, 100, 15)
x2 = x1
t.test(x1, x2, var.eq=T)
Two Sample t-test
data: x1 and x2
t = 0, df = 2e+05, p-value = 1
alternative hypothesis: true difference in means is not equal to 0
95 percent confidence interval:
-0.1316593 0.1316593
sample estimates:
mean of x mean of y
99.96403 99.96403
$endgroup$
It is difficult to have a cogent philosophical discussion about things
that have 0 probability of happening. So I will show you some examples
that relate to your question.
If you have two enormous independent samples from the same distribution,
then both samples will still have some variability, the pooled 2-sample t statistic will
be near, but not exactly 0, the P-value will be distributed as
$mathsfUnif(0,1),$ and the 95% confidence interval will be very short
and centered very near $0.$
An example of one such dataset and t test:
set.seed(902)
x1 = rnorm(10^5, 100, 15)
x2 = rnorm(10^5, 100, 15)
t.test(x1, x2, var.eq=T)
Two Sample t-test
data: x1 and x2
t = -0.41372, df = 2e+05, p-value = 0.6791
alternative hypothesis: true difference in means is not equal to 0
95 percent confidence interval:
-0.1591659 0.1036827
sample estimates:
mean of x mean of y
99.96403 99.99177
Here are summarized results from 10,000 such situations. First, the distribution of P-values.
set.seed(2019)
pv = replicate(10^4,
t.test(rnorm(10^5,100,15),rnorm(10^5,100,15),var.eq=T)$p.val)
mean(pv)
[1] 0.5007066 # aprx 1/2
hist(pv, prob=T, col="skyblue2", main="Simulated P-values")
curve(dunif(x), add=T, col="red", lwd=2, n=10001)
Next the test statistic:
set.seed(2019) # same seed as above, so same 10^4 datasets
st = replicate(10^4,
t.test(rnorm(10^5,100,15),rnorm(10^5,100,15),var.eq=T)$stat)
mean(st)
[1] 0.002810332 # aprx 0
hist(st, prob=T, col="skyblue2", main="Simulated P-values")
curve(dt(x, df=2e+05), add=T, col="red", lwd=2, n=10001)
And so on for the width of the CI.
set.seed(2019)
w.ci = replicate(10^4,
diff(t.test(rnorm(10^5,100,15),
rnorm(10^5,100,15),var.eq=T)$conf.int))
mean(w.ci)
[1] 0.2629603
It is almost impossible to get a
P-value of unity doing an exact test with continuous data, where
assumptions are met. So much so, that a wise statistician will ponder
what might have gone wrong upon seeing a P-value of 1.
For example, you might give the software two identical large samples.
The programming will carry on as if these are two independent samples, and
give strange results. But even then the CI will not be of 0 width.
set.seed(902)
x1 = rnorm(10^5, 100, 15)
x2 = x1
t.test(x1, x2, var.eq=T)
Two Sample t-test
data: x1 and x2
t = 0, df = 2e+05, p-value = 1
alternative hypothesis: true difference in means is not equal to 0
95 percent confidence interval:
-0.1316593 0.1316593
sample estimates:
mean of x mean of y
99.96403 99.96403
edited 3 hours ago
answered 4 hours ago
BruceETBruceET
13.8k1 gold badge9 silver badges28 bronze badges
13.8k1 gold badge9 silver badges28 bronze badges
add a comment |
add a comment |
Thanks for contributing an answer to Cross Validated!
- Please be sure to answer the question. Provide details and share your research!
But avoid …
- Asking for help, clarification, or responding to other answers.
- Making statements based on opinion; back them up with references or personal experience.
Use MathJax to format equations. MathJax reference.
To learn more, see our tips on writing great answers.
Sign up or log in
StackExchange.ready(function ()
StackExchange.helpers.onClickDraftSave('#login-link');
);
Sign up using Google
Sign up using Facebook
Sign up using Email and Password
Post as a guest
Required, but never shown
StackExchange.ready(
function ()
StackExchange.openid.initPostLogin('.new-post-login', 'https%3a%2f%2fstats.stackexchange.com%2fquestions%2f424656%2fif-p-value-is-exactly-1-1-0000000-what-are-the-confidence-interval-limits%23new-answer', 'question_page');
);
Post as a guest
Required, but never shown
Sign up or log in
StackExchange.ready(function ()
StackExchange.helpers.onClickDraftSave('#login-link');
);
Sign up using Google
Sign up using Facebook
Sign up using Email and Password
Post as a guest
Required, but never shown
Sign up or log in
StackExchange.ready(function ()
StackExchange.helpers.onClickDraftSave('#login-link');
);
Sign up using Google
Sign up using Facebook
Sign up using Email and Password
Post as a guest
Required, but never shown
Sign up or log in
StackExchange.ready(function ()
StackExchange.helpers.onClickDraftSave('#login-link');
);
Sign up using Google
Sign up using Facebook
Sign up using Email and Password
Sign up using Google
Sign up using Facebook
Sign up using Email and Password
Post as a guest
Required, but never shown
Required, but never shown
Required, but never shown
Required, but never shown
Required, but never shown
Required, but never shown
Required, but never shown
Required, but never shown
Required, but never shown
ep a1,nR8,EhftIYwKaC3FgU0QzVp11BqY
$begingroup$
I'd say that this was already answered in here stats.stackexchange.com/questions/275677/…, but I'm not insisting on it.
$endgroup$
– Tim♦
8 hours ago
$begingroup$
If p was exactly unity, then it would be exactly 100% risky to reject the null hypothesis, whatever it was. So whatever lead to p = 1 would have given no reason whatsoever to doubt the null hypothesis.
$endgroup$
– Ed V
8 hours ago
$begingroup$
I’m having trouble coming up with a way to get $p=1$ with positive population variances.
$endgroup$
– Dave
8 hours ago
$begingroup$
I'm no expert, but my understanding of the logic of hypothesis testing is that $pRightarrow q$, where $p$ is some condition on the p-value and $q$ is rejecting the null hypothesis. You CANNOT conclude that $sim pRightarrow sim q$, No matter how confident you are about $sim p$, which I guess answers your question.
$endgroup$
– idnavid
8 hours ago
$begingroup$
The CI will be symmetric around $0$ but it won't be zero because we would still have a finite sample effect. As $n$ approach infinity we would get the CI becoming narrower and narrower but that is not due to the difference being zero. Note that the "narrowing" of the CI would be true even if $mu_A neq mu_B$.
$endgroup$
– usεr11852
7 hours ago