How do I deal with large amout missing values in a data set without dropping them?Imputation of missing response variablesHow to deal with non-numeric missing values with pythonHow do I impute missing values of a dataset with little pairwise correction using regressionIn real clinical diagnostic data set how can we know the “true label” of a patient?A data set with missing values in multiple variablesMissing values in a large data setShould I impute Missing Laboratory Data? - Confused about MAR-MNARFollow up medical study with missing dataWill I break my model if I replace missing values with `Unknown`?dealing with missing values on train set Or combined set
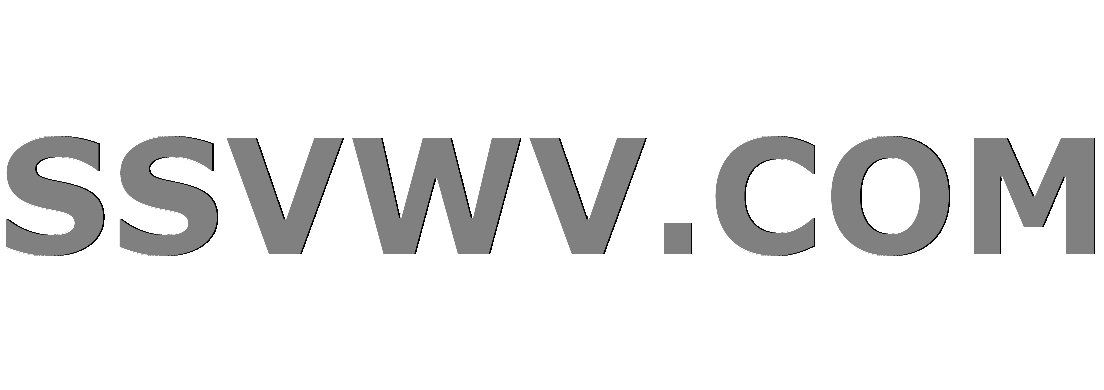
Multi tool use
Why do proponents of guns oppose gun competency tests?
What is an air conditioner compressor hard start kit and how does it work?
Why private jets such as GulfStream ones fly higher than other civil jets?
split large formula in align
Can I enter Switzerland with only my London Driver's License?
If someone else uploads my GPL'd code to Github without my permission, is that a copyright violation?
How can I perform a deterministic physics simulation?
Is an "are" omitted in this sentence
What prevents ads from reading my password as I type it?
Which genus do I use for neutral expressions in German?
Is space radiation a risk for space film photography, and how is this prevented?
Does a humanoid possessed by a ghost register as undead to a paladin's Divine Sense?
How to make attic easier to traverse?
Could an areostationary satellite help locate asteroids?
How important is it to have a spot meter on the light meter?
Ubuntu show wrong disk sizes, how to solve it?
Is there a way to say "double + any number" in German?
Whats the difference between <processors> and <pipelines> in Sitecore configuration?
The Game of the Century - why didn't Byrne take the rook after he forked Fischer?
Pronouns when writing from the point of view of a robot
What's going on with an item that starts with an hbox?
Can attackers change the public key of certificate during the SSL handshake
Premier League simulation
How to switch an 80286 from protected to real mode?
How do I deal with large amout missing values in a data set without dropping them?
Imputation of missing response variablesHow to deal with non-numeric missing values with pythonHow do I impute missing values of a dataset with little pairwise correction using regressionIn real clinical diagnostic data set how can we know the “true label” of a patient?A data set with missing values in multiple variablesMissing values in a large data setShould I impute Missing Laboratory Data? - Confused about MAR-MNARFollow up medical study with missing dataWill I break my model if I replace missing values with `Unknown`?dealing with missing values on train set Or combined set
.everyoneloves__top-leaderboard:empty,.everyoneloves__mid-leaderboard:empty,.everyoneloves__bot-mid-leaderboard:empty margin-bottom:0;
$begingroup$
I am trying to build a binary classification model which predicts whether a patient would me infected with a certain disease at the the end of his hospital stay or not. The features that I have are results of different standard medical tests. But the issue is almost all of these results have around 60% - 80% missing values as not all the tests are relevant for all the patients. So how do I deal with the missing values as dropping them is not an option here. Also since the medical test results lie on scale ranging from low to high, should i converted them to categorical variable with High, Low, Medium, Null (for missing data), based on the standard medical test ranges? Or would it helpful to replace with any of the central tendencies?
classification python missing-data feature-engineering
New contributor
Krantikari is a new contributor to this site. Take care in asking for clarification, commenting, and answering.
Check out our Code of Conduct.
$endgroup$
add a comment |
$begingroup$
I am trying to build a binary classification model which predicts whether a patient would me infected with a certain disease at the the end of his hospital stay or not. The features that I have are results of different standard medical tests. But the issue is almost all of these results have around 60% - 80% missing values as not all the tests are relevant for all the patients. So how do I deal with the missing values as dropping them is not an option here. Also since the medical test results lie on scale ranging from low to high, should i converted them to categorical variable with High, Low, Medium, Null (for missing data), based on the standard medical test ranges? Or would it helpful to replace with any of the central tendencies?
classification python missing-data feature-engineering
New contributor
Krantikari is a new contributor to this site. Take care in asking for clarification, commenting, and answering.
Check out our Code of Conduct.
$endgroup$
add a comment |
$begingroup$
I am trying to build a binary classification model which predicts whether a patient would me infected with a certain disease at the the end of his hospital stay or not. The features that I have are results of different standard medical tests. But the issue is almost all of these results have around 60% - 80% missing values as not all the tests are relevant for all the patients. So how do I deal with the missing values as dropping them is not an option here. Also since the medical test results lie on scale ranging from low to high, should i converted them to categorical variable with High, Low, Medium, Null (for missing data), based on the standard medical test ranges? Or would it helpful to replace with any of the central tendencies?
classification python missing-data feature-engineering
New contributor
Krantikari is a new contributor to this site. Take care in asking for clarification, commenting, and answering.
Check out our Code of Conduct.
$endgroup$
I am trying to build a binary classification model which predicts whether a patient would me infected with a certain disease at the the end of his hospital stay or not. The features that I have are results of different standard medical tests. But the issue is almost all of these results have around 60% - 80% missing values as not all the tests are relevant for all the patients. So how do I deal with the missing values as dropping them is not an option here. Also since the medical test results lie on scale ranging from low to high, should i converted them to categorical variable with High, Low, Medium, Null (for missing data), based on the standard medical test ranges? Or would it helpful to replace with any of the central tendencies?
classification python missing-data feature-engineering
classification python missing-data feature-engineering
New contributor
Krantikari is a new contributor to this site. Take care in asking for clarification, commenting, and answering.
Check out our Code of Conduct.
New contributor
Krantikari is a new contributor to this site. Take care in asking for clarification, commenting, and answering.
Check out our Code of Conduct.
New contributor
Krantikari is a new contributor to this site. Take care in asking for clarification, commenting, and answering.
Check out our Code of Conduct.
asked 8 hours ago
KrantikariKrantikari
111 bronze badge
111 bronze badge
New contributor
Krantikari is a new contributor to this site. Take care in asking for clarification, commenting, and answering.
Check out our Code of Conduct.
New contributor
Krantikari is a new contributor to this site. Take care in asking for clarification, commenting, and answering.
Check out our Code of Conduct.
add a comment |
add a comment |
2 Answers
2
active
oldest
votes
$begingroup$
Because NA values are informative for your dataset, you don't want to drop NAs or impute values. If a patient doesn't get an X-ray, they probably didn't break a bone.
So you want to learn from NA values. A common approach is to add an indicator column for NA values.
New contributor
damerdji is a new contributor to this site. Take care in asking for clarification, commenting, and answering.
Check out our Code of Conduct.
$endgroup$
$begingroup$
OK but how do I replace the NA values in the original column?
$endgroup$
– Krantikari
7 hours ago
1
$begingroup$
You don't want to use values in the range of possible test results. I'm guessing -999 will work, but this is data-dependent.
$endgroup$
– damerdji
7 hours ago
1
$begingroup$
I should add that if you make that change, only nonlinear models will learn effectively.
$endgroup$
– damerdji
7 hours ago
$begingroup$
Tree based models should work fine right?
$endgroup$
– Krantikari
7 hours ago
$begingroup$
Yup! Tree-based models are nonlinear.
$endgroup$
– damerdji
7 hours ago
add a comment |
$begingroup$
A linear mixed effects model would allow you to have individuals with missing data and not need to convert everything over to categories. If ever you have a continuous variable, use it as a continuum if at all possible.
Here is a link to a paper that explains more about why. It is not just for psychologists, the same applies because the arguments are based on math, not opinion. https://www.researchgate.net/publication/282351876_The_problem_with_categorical_thinking_by_psychologists
If you have data on a bunch of known cases you can use to build the model, use a logistic generalized linear mixed-effects model aka logistic GLMM. In R it is in the lme4 library and uses GLMER for its call (Generalized Linear Mixed Effects Regression). You may also want to look into signal detection theory as it may help you out here. With a logistic GLMM you can use an individual patient's information in the model and it will give you the odds of them having/not having the outcome. Just be careful to add only relevant variables to your model. If there are too many predictors your model will not generalize well to new patients that were not used to fit the model. To remedy this, if you have enough data, split it at random into two data sets, fit the model on one data set and then see how well it predicts another data set by comparing the Akaike Information Criterion and Bayesian Information Criterion. Bootstrapping may also help.
GLMMs and LMMs in general deal very will with missing data. Unlike a traditional logistic regression, LMMs do not have the assumption of equal cell sizes. Don't be fooled, if someone says that ANOVA/regression is robust to violations of its assumptions, especially if the cell sizes are unequal. They haven't done their homework and are just parroting what they heard in grad school. the math on that is clear.
$endgroup$
add a comment |
Your Answer
StackExchange.ready(function()
var channelOptions =
tags: "".split(" "),
id: "65"
;
initTagRenderer("".split(" "), "".split(" "), channelOptions);
StackExchange.using("externalEditor", function()
// Have to fire editor after snippets, if snippets enabled
if (StackExchange.settings.snippets.snippetsEnabled)
StackExchange.using("snippets", function()
createEditor();
);
else
createEditor();
);
function createEditor()
StackExchange.prepareEditor(
heartbeatType: 'answer',
autoActivateHeartbeat: false,
convertImagesToLinks: false,
noModals: true,
showLowRepImageUploadWarning: true,
reputationToPostImages: null,
bindNavPrevention: true,
postfix: "",
imageUploader:
brandingHtml: "Powered by u003ca class="icon-imgur-white" href="https://imgur.com/"u003eu003c/au003e",
contentPolicyHtml: "User contributions licensed under u003ca href="https://creativecommons.org/licenses/by-sa/3.0/"u003ecc by-sa 3.0 with attribution requiredu003c/au003e u003ca href="https://stackoverflow.com/legal/content-policy"u003e(content policy)u003c/au003e",
allowUrls: true
,
onDemand: true,
discardSelector: ".discard-answer"
,immediatelyShowMarkdownHelp:true
);
);
Krantikari is a new contributor. Be nice, and check out our Code of Conduct.
Sign up or log in
StackExchange.ready(function ()
StackExchange.helpers.onClickDraftSave('#login-link');
);
Sign up using Google
Sign up using Facebook
Sign up using Email and Password
Post as a guest
Required, but never shown
StackExchange.ready(
function ()
StackExchange.openid.initPostLogin('.new-post-login', 'https%3a%2f%2fstats.stackexchange.com%2fquestions%2f420949%2fhow-do-i-deal-with-large-amout-missing-values-in-a-data-set-without-dropping-the%23new-answer', 'question_page');
);
Post as a guest
Required, but never shown
2 Answers
2
active
oldest
votes
2 Answers
2
active
oldest
votes
active
oldest
votes
active
oldest
votes
$begingroup$
Because NA values are informative for your dataset, you don't want to drop NAs or impute values. If a patient doesn't get an X-ray, they probably didn't break a bone.
So you want to learn from NA values. A common approach is to add an indicator column for NA values.
New contributor
damerdji is a new contributor to this site. Take care in asking for clarification, commenting, and answering.
Check out our Code of Conduct.
$endgroup$
$begingroup$
OK but how do I replace the NA values in the original column?
$endgroup$
– Krantikari
7 hours ago
1
$begingroup$
You don't want to use values in the range of possible test results. I'm guessing -999 will work, but this is data-dependent.
$endgroup$
– damerdji
7 hours ago
1
$begingroup$
I should add that if you make that change, only nonlinear models will learn effectively.
$endgroup$
– damerdji
7 hours ago
$begingroup$
Tree based models should work fine right?
$endgroup$
– Krantikari
7 hours ago
$begingroup$
Yup! Tree-based models are nonlinear.
$endgroup$
– damerdji
7 hours ago
add a comment |
$begingroup$
Because NA values are informative for your dataset, you don't want to drop NAs or impute values. If a patient doesn't get an X-ray, they probably didn't break a bone.
So you want to learn from NA values. A common approach is to add an indicator column for NA values.
New contributor
damerdji is a new contributor to this site. Take care in asking for clarification, commenting, and answering.
Check out our Code of Conduct.
$endgroup$
$begingroup$
OK but how do I replace the NA values in the original column?
$endgroup$
– Krantikari
7 hours ago
1
$begingroup$
You don't want to use values in the range of possible test results. I'm guessing -999 will work, but this is data-dependent.
$endgroup$
– damerdji
7 hours ago
1
$begingroup$
I should add that if you make that change, only nonlinear models will learn effectively.
$endgroup$
– damerdji
7 hours ago
$begingroup$
Tree based models should work fine right?
$endgroup$
– Krantikari
7 hours ago
$begingroup$
Yup! Tree-based models are nonlinear.
$endgroup$
– damerdji
7 hours ago
add a comment |
$begingroup$
Because NA values are informative for your dataset, you don't want to drop NAs or impute values. If a patient doesn't get an X-ray, they probably didn't break a bone.
So you want to learn from NA values. A common approach is to add an indicator column for NA values.
New contributor
damerdji is a new contributor to this site. Take care in asking for clarification, commenting, and answering.
Check out our Code of Conduct.
$endgroup$
Because NA values are informative for your dataset, you don't want to drop NAs or impute values. If a patient doesn't get an X-ray, they probably didn't break a bone.
So you want to learn from NA values. A common approach is to add an indicator column for NA values.
New contributor
damerdji is a new contributor to this site. Take care in asking for clarification, commenting, and answering.
Check out our Code of Conduct.
New contributor
damerdji is a new contributor to this site. Take care in asking for clarification, commenting, and answering.
Check out our Code of Conduct.
answered 7 hours ago


damerdjidamerdji
714 bronze badges
714 bronze badges
New contributor
damerdji is a new contributor to this site. Take care in asking for clarification, commenting, and answering.
Check out our Code of Conduct.
New contributor
damerdji is a new contributor to this site. Take care in asking for clarification, commenting, and answering.
Check out our Code of Conduct.
$begingroup$
OK but how do I replace the NA values in the original column?
$endgroup$
– Krantikari
7 hours ago
1
$begingroup$
You don't want to use values in the range of possible test results. I'm guessing -999 will work, but this is data-dependent.
$endgroup$
– damerdji
7 hours ago
1
$begingroup$
I should add that if you make that change, only nonlinear models will learn effectively.
$endgroup$
– damerdji
7 hours ago
$begingroup$
Tree based models should work fine right?
$endgroup$
– Krantikari
7 hours ago
$begingroup$
Yup! Tree-based models are nonlinear.
$endgroup$
– damerdji
7 hours ago
add a comment |
$begingroup$
OK but how do I replace the NA values in the original column?
$endgroup$
– Krantikari
7 hours ago
1
$begingroup$
You don't want to use values in the range of possible test results. I'm guessing -999 will work, but this is data-dependent.
$endgroup$
– damerdji
7 hours ago
1
$begingroup$
I should add that if you make that change, only nonlinear models will learn effectively.
$endgroup$
– damerdji
7 hours ago
$begingroup$
Tree based models should work fine right?
$endgroup$
– Krantikari
7 hours ago
$begingroup$
Yup! Tree-based models are nonlinear.
$endgroup$
– damerdji
7 hours ago
$begingroup$
OK but how do I replace the NA values in the original column?
$endgroup$
– Krantikari
7 hours ago
$begingroup$
OK but how do I replace the NA values in the original column?
$endgroup$
– Krantikari
7 hours ago
1
1
$begingroup$
You don't want to use values in the range of possible test results. I'm guessing -999 will work, but this is data-dependent.
$endgroup$
– damerdji
7 hours ago
$begingroup$
You don't want to use values in the range of possible test results. I'm guessing -999 will work, but this is data-dependent.
$endgroup$
– damerdji
7 hours ago
1
1
$begingroup$
I should add that if you make that change, only nonlinear models will learn effectively.
$endgroup$
– damerdji
7 hours ago
$begingroup$
I should add that if you make that change, only nonlinear models will learn effectively.
$endgroup$
– damerdji
7 hours ago
$begingroup$
Tree based models should work fine right?
$endgroup$
– Krantikari
7 hours ago
$begingroup$
Tree based models should work fine right?
$endgroup$
– Krantikari
7 hours ago
$begingroup$
Yup! Tree-based models are nonlinear.
$endgroup$
– damerdji
7 hours ago
$begingroup$
Yup! Tree-based models are nonlinear.
$endgroup$
– damerdji
7 hours ago
add a comment |
$begingroup$
A linear mixed effects model would allow you to have individuals with missing data and not need to convert everything over to categories. If ever you have a continuous variable, use it as a continuum if at all possible.
Here is a link to a paper that explains more about why. It is not just for psychologists, the same applies because the arguments are based on math, not opinion. https://www.researchgate.net/publication/282351876_The_problem_with_categorical_thinking_by_psychologists
If you have data on a bunch of known cases you can use to build the model, use a logistic generalized linear mixed-effects model aka logistic GLMM. In R it is in the lme4 library and uses GLMER for its call (Generalized Linear Mixed Effects Regression). You may also want to look into signal detection theory as it may help you out here. With a logistic GLMM you can use an individual patient's information in the model and it will give you the odds of them having/not having the outcome. Just be careful to add only relevant variables to your model. If there are too many predictors your model will not generalize well to new patients that were not used to fit the model. To remedy this, if you have enough data, split it at random into two data sets, fit the model on one data set and then see how well it predicts another data set by comparing the Akaike Information Criterion and Bayesian Information Criterion. Bootstrapping may also help.
GLMMs and LMMs in general deal very will with missing data. Unlike a traditional logistic regression, LMMs do not have the assumption of equal cell sizes. Don't be fooled, if someone says that ANOVA/regression is robust to violations of its assumptions, especially if the cell sizes are unequal. They haven't done their homework and are just parroting what they heard in grad school. the math on that is clear.
$endgroup$
add a comment |
$begingroup$
A linear mixed effects model would allow you to have individuals with missing data and not need to convert everything over to categories. If ever you have a continuous variable, use it as a continuum if at all possible.
Here is a link to a paper that explains more about why. It is not just for psychologists, the same applies because the arguments are based on math, not opinion. https://www.researchgate.net/publication/282351876_The_problem_with_categorical_thinking_by_psychologists
If you have data on a bunch of known cases you can use to build the model, use a logistic generalized linear mixed-effects model aka logistic GLMM. In R it is in the lme4 library and uses GLMER for its call (Generalized Linear Mixed Effects Regression). You may also want to look into signal detection theory as it may help you out here. With a logistic GLMM you can use an individual patient's information in the model and it will give you the odds of them having/not having the outcome. Just be careful to add only relevant variables to your model. If there are too many predictors your model will not generalize well to new patients that were not used to fit the model. To remedy this, if you have enough data, split it at random into two data sets, fit the model on one data set and then see how well it predicts another data set by comparing the Akaike Information Criterion and Bayesian Information Criterion. Bootstrapping may also help.
GLMMs and LMMs in general deal very will with missing data. Unlike a traditional logistic regression, LMMs do not have the assumption of equal cell sizes. Don't be fooled, if someone says that ANOVA/regression is robust to violations of its assumptions, especially if the cell sizes are unequal. They haven't done their homework and are just parroting what they heard in grad school. the math on that is clear.
$endgroup$
add a comment |
$begingroup$
A linear mixed effects model would allow you to have individuals with missing data and not need to convert everything over to categories. If ever you have a continuous variable, use it as a continuum if at all possible.
Here is a link to a paper that explains more about why. It is not just for psychologists, the same applies because the arguments are based on math, not opinion. https://www.researchgate.net/publication/282351876_The_problem_with_categorical_thinking_by_psychologists
If you have data on a bunch of known cases you can use to build the model, use a logistic generalized linear mixed-effects model aka logistic GLMM. In R it is in the lme4 library and uses GLMER for its call (Generalized Linear Mixed Effects Regression). You may also want to look into signal detection theory as it may help you out here. With a logistic GLMM you can use an individual patient's information in the model and it will give you the odds of them having/not having the outcome. Just be careful to add only relevant variables to your model. If there are too many predictors your model will not generalize well to new patients that were not used to fit the model. To remedy this, if you have enough data, split it at random into two data sets, fit the model on one data set and then see how well it predicts another data set by comparing the Akaike Information Criterion and Bayesian Information Criterion. Bootstrapping may also help.
GLMMs and LMMs in general deal very will with missing data. Unlike a traditional logistic regression, LMMs do not have the assumption of equal cell sizes. Don't be fooled, if someone says that ANOVA/regression is robust to violations of its assumptions, especially if the cell sizes are unequal. They haven't done their homework and are just parroting what they heard in grad school. the math on that is clear.
$endgroup$
A linear mixed effects model would allow you to have individuals with missing data and not need to convert everything over to categories. If ever you have a continuous variable, use it as a continuum if at all possible.
Here is a link to a paper that explains more about why. It is not just for psychologists, the same applies because the arguments are based on math, not opinion. https://www.researchgate.net/publication/282351876_The_problem_with_categorical_thinking_by_psychologists
If you have data on a bunch of known cases you can use to build the model, use a logistic generalized linear mixed-effects model aka logistic GLMM. In R it is in the lme4 library and uses GLMER for its call (Generalized Linear Mixed Effects Regression). You may also want to look into signal detection theory as it may help you out here. With a logistic GLMM you can use an individual patient's information in the model and it will give you the odds of them having/not having the outcome. Just be careful to add only relevant variables to your model. If there are too many predictors your model will not generalize well to new patients that were not used to fit the model. To remedy this, if you have enough data, split it at random into two data sets, fit the model on one data set and then see how well it predicts another data set by comparing the Akaike Information Criterion and Bayesian Information Criterion. Bootstrapping may also help.
GLMMs and LMMs in general deal very will with missing data. Unlike a traditional logistic regression, LMMs do not have the assumption of equal cell sizes. Don't be fooled, if someone says that ANOVA/regression is robust to violations of its assumptions, especially if the cell sizes are unequal. They haven't done their homework and are just parroting what they heard in grad school. the math on that is clear.
edited 4 hours ago
answered 7 hours ago


Thomas WukitschThomas Wukitsch
565 bronze badges
565 bronze badges
add a comment |
add a comment |
Krantikari is a new contributor. Be nice, and check out our Code of Conduct.
Krantikari is a new contributor. Be nice, and check out our Code of Conduct.
Krantikari is a new contributor. Be nice, and check out our Code of Conduct.
Krantikari is a new contributor. Be nice, and check out our Code of Conduct.
Thanks for contributing an answer to Cross Validated!
- Please be sure to answer the question. Provide details and share your research!
But avoid …
- Asking for help, clarification, or responding to other answers.
- Making statements based on opinion; back them up with references or personal experience.
Use MathJax to format equations. MathJax reference.
To learn more, see our tips on writing great answers.
Sign up or log in
StackExchange.ready(function ()
StackExchange.helpers.onClickDraftSave('#login-link');
);
Sign up using Google
Sign up using Facebook
Sign up using Email and Password
Post as a guest
Required, but never shown
StackExchange.ready(
function ()
StackExchange.openid.initPostLogin('.new-post-login', 'https%3a%2f%2fstats.stackexchange.com%2fquestions%2f420949%2fhow-do-i-deal-with-large-amout-missing-values-in-a-data-set-without-dropping-the%23new-answer', 'question_page');
);
Post as a guest
Required, but never shown
Sign up or log in
StackExchange.ready(function ()
StackExchange.helpers.onClickDraftSave('#login-link');
);
Sign up using Google
Sign up using Facebook
Sign up using Email and Password
Post as a guest
Required, but never shown
Sign up or log in
StackExchange.ready(function ()
StackExchange.helpers.onClickDraftSave('#login-link');
);
Sign up using Google
Sign up using Facebook
Sign up using Email and Password
Post as a guest
Required, but never shown
Sign up or log in
StackExchange.ready(function ()
StackExchange.helpers.onClickDraftSave('#login-link');
);
Sign up using Google
Sign up using Facebook
Sign up using Email and Password
Sign up using Google
Sign up using Facebook
Sign up using Email and Password
Post as a guest
Required, but never shown
Required, but never shown
Required, but never shown
Required, but never shown
Required, but never shown
Required, but never shown
Required, but never shown
Required, but never shown
Required, but never shown
UfEXLJPqluf AMs27Gd1,Q,6UUqsQ6hbIm76mEKWwA39H7bW,lqPesOgHnyNQ IV4XhxZtT,AD8xpjO